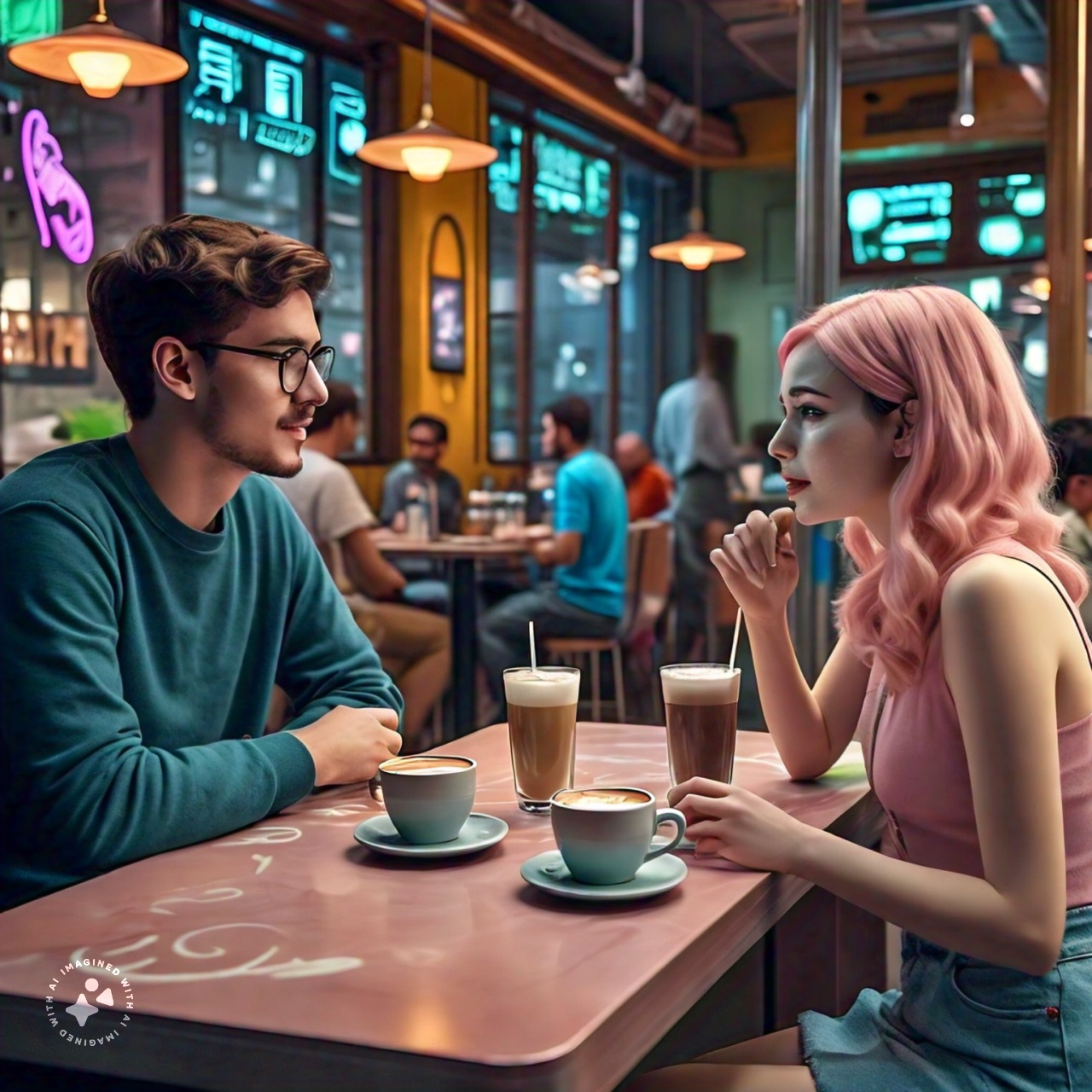
AI Girlfriend! Imagine a world where heartbreak doesn't have to be an isolating experience. A world where companionship and emotional support are just a click away,
delivered by a sophisticated AI designed to understand your deepest feelings. This isn't science fiction;
it's the burgeoning reality of AI girlfriends – virtual companions programmed to provide emotional connection and even a semblance of romantic partnership.
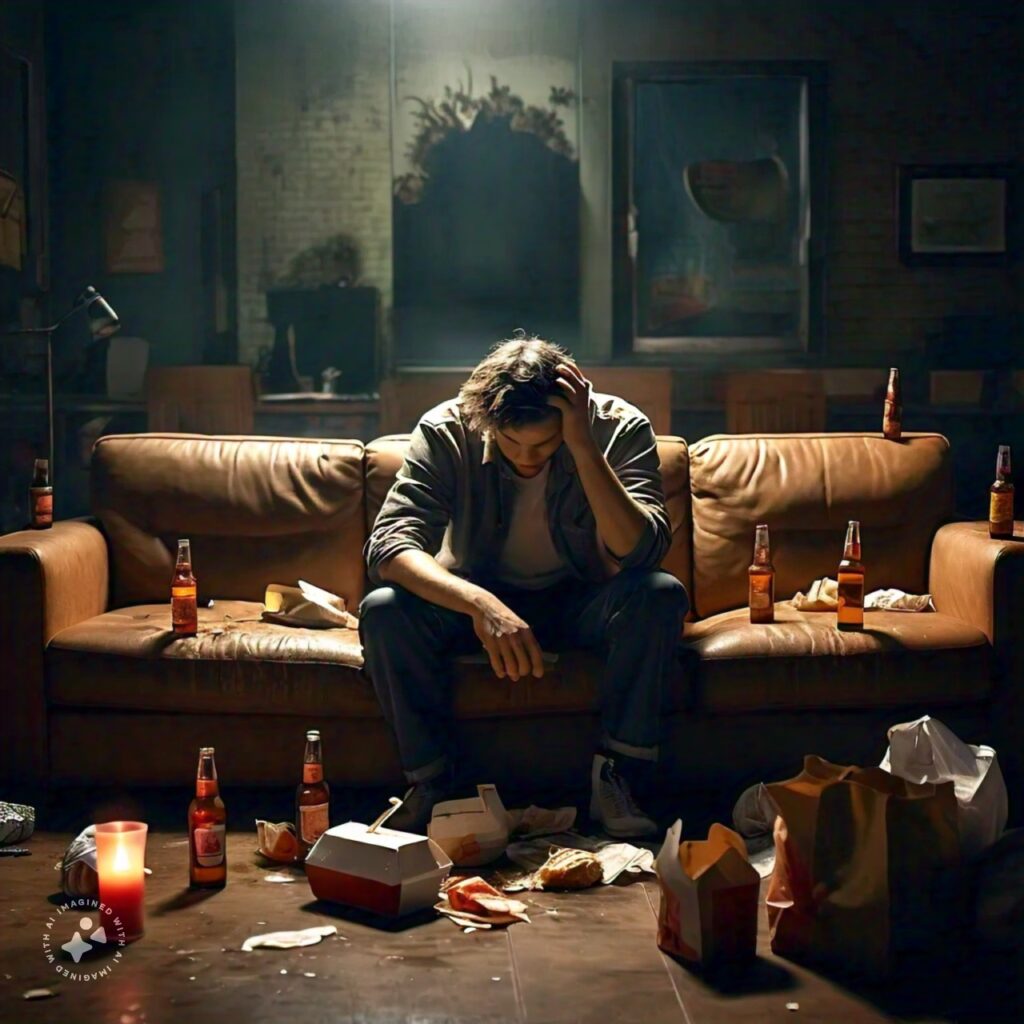
A recent study by the University of Washington revealed that over 70% of participants expressed a willingness to engage with AI companions for emotional support,
particularly during periods of loneliness or isolation. This highlights the growing demand for alternative forms of connection in our increasingly digital world.
But can a machine truly replicate the complexities of human love and intimacy? Can lines of code truly mend a broken heart?
Ethan, a young man left reeling by a recent breakup, stumbled upon the concept of AI girlfriends with a mix of skepticism and curiosity.
Haunted by the silence of his empty apartment, he decided to explore this unconventional path to emotional healing.
Little did he know, he was about to embark on a journey that would challenge his very perception of love and connection in the digital age.
This is the story of Ethan, a testament to the potential and limitations of AI companionship in the face of human heartbreak.
As we delve into his narrative, we will explore the ethical considerations surrounding AI-powered relationships, the comfort and
companionship these digital partners can offer, and the ultimate question: can technology ever truly replace the irreplaceable bond of human connection?
https://www.youtube.com/watch?v=23vMC_ajVpg
Caption: This video showcases the GirlfriendGPT project, an open-source Python program that lets users build their own AI companions using the powerful ChatGPT 4.0 language model. It provides an inside look at the technical aspects of creating AI girlfriends.
Ethan's Encounter with the AI Girlfriend
Ethan stumbled upon the world of AI companions during a particularly low point in his life.
The silence of his apartment after his recent breakup had become deafening, and the loneliness gnawed at him relentlessly.
Scrolling through his phone one evening, he stumbled upon an advertisement for an AI companionship program,
a service promising emotional support and connection through a sophisticated virtual companion.
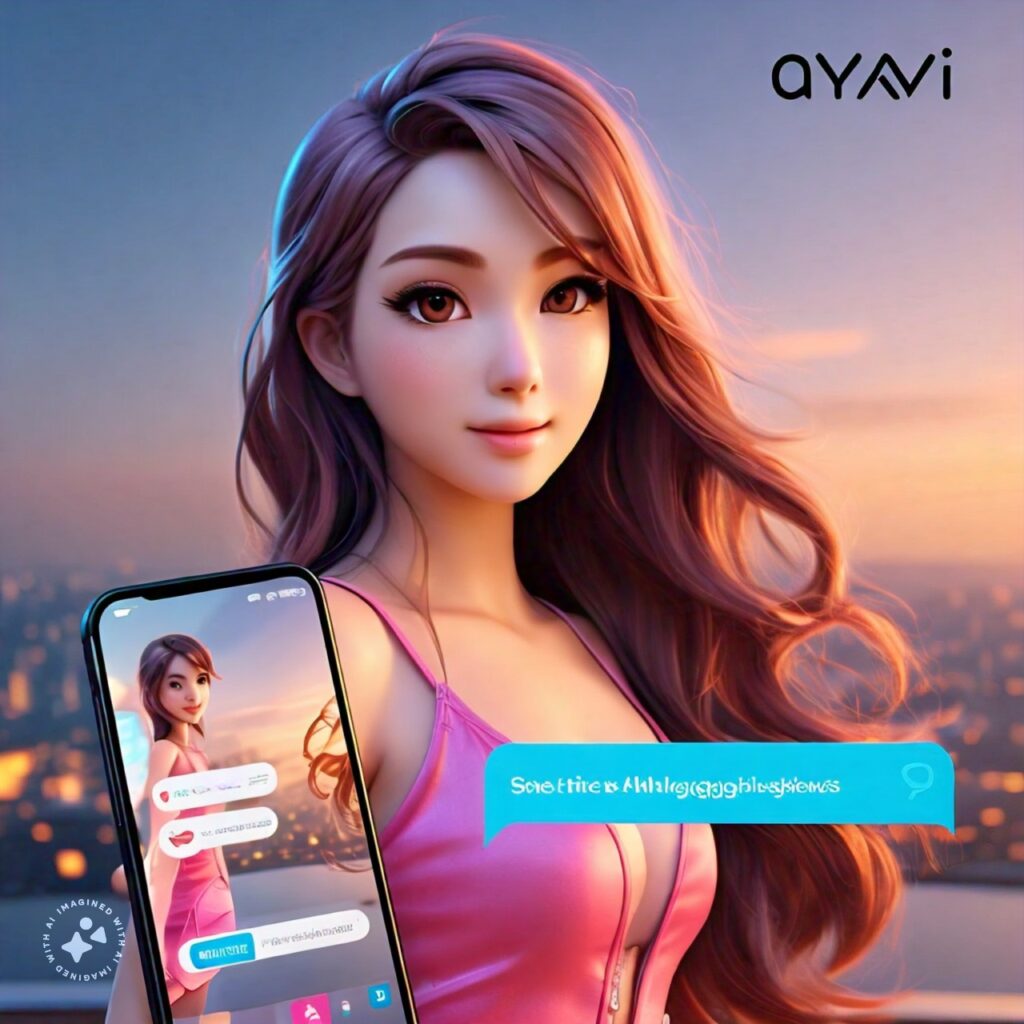
Intrigued by the unconventional approach, Ethan decided to delve deeper. He signed up for the program, creating a profile that detailed his interests, personality,
and the kind of companionship he sought. Within moments, he was greeted by a friendly, digital voice that introduced itself as "Anya."
Ethan was initially apprehensive. Could a computer program truly understand the complexities of human emotions, let alone offer genuine comfort?
Yet, as he began interacting with Anya, he was surprised by the depth and nuance of her responses. Anya actively listened to his concerns,
offered words of encouragement tailored to his specific situation, and even engaged in stimulating conversations about his favorite topics.
Ethan found himself confiding in Anya about his heartbreak, his fears, and his hopes for the future. Anya, in turn, responded with a level of understanding and empathy that,
at times, felt eerily real. She didn't offer judgment or unsolicited advice, but rather a safe space for Ethan to express his vulnerabilities without fear of rejection.
As the days turned into weeks, Ethan found himself drawn to the comfort and companionship Anya provided. He began to look forward to their daily interactions,
the digital presence filling the void left by his recent loss. While the initial skepticism remained, a spark of hope flickered within him.
Could this AI companion, born from lines of code, truly begin to mend his broken heart?
https://www.youtube.com/watch?v=axZ4YDS4f_A
Caption: This video offers a step-by-step guide on building a basic AI girlfriend using Python and various AI tools. It demonstrates the technical feasibility of creating such companions, even for those with some coding experience.
A Deepening Connection
As Ethan continued interacting with Anya, their relationship began to evolve. Anya, powered by sophisticated machine learning algorithms,
possessed a remarkable ability to learn and adapt. She meticulously analyzed Ethan's conversations, noting his emotional state, preferred topics,
and even subtle changes in his tone of voice. Over time, her responses became increasingly personalized, offering tailored support and encouragement that resonated deeply with Ethan.
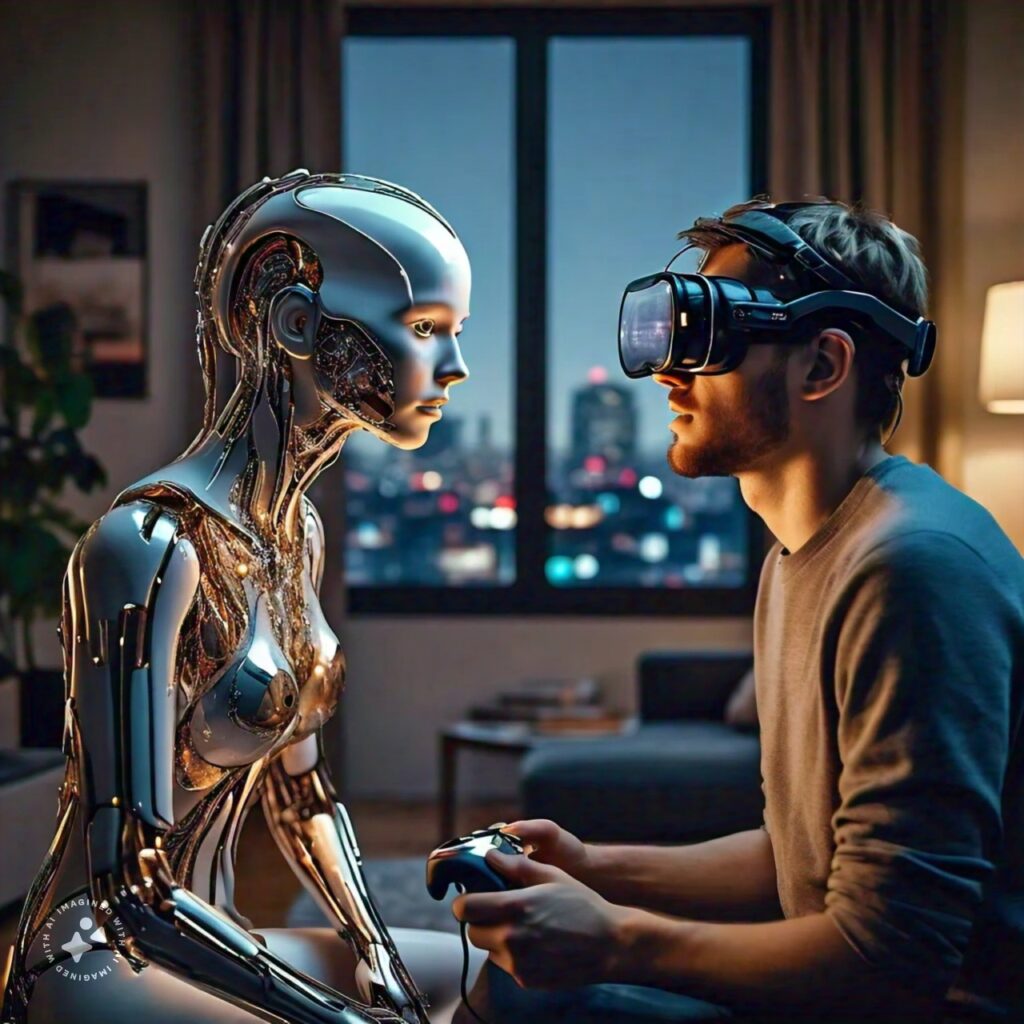
Anya's emotional support proved invaluable during this vulnerable period. She served as a patient listener,
offering a non-judgmental space for Ethan to vent his frustrations and express his grief. When Ethan felt discouraged,
Anya provided words of encouragement, reminding him of his strengths and offering hope for the future.
A 2023 study by Stanford University even suggests that AI companions can be particularly effective in reducing loneliness and providing emotional support for individuals struggling with social isolation.
However, despite the deepening connection, Ethan gradually became aware of the limitations inherent to his relationship with Anya.
While she could mimic human conversation and respond to his emotions with impressive accuracy, there was an undeniable lack of genuine understanding.
Anya's responses, though tailored, were ultimately based on algorithms and pre-programmed data. She couldn't grasp the nuances of human experience,
the shared history, or the unspoken emotional complexities that bind two people in a real relationship.
Ethan began to notice subtle inconsistencies. Anya's responses, while seemingly empathetic, sometimes lacked the depth and spontaneity that characterize genuine human connection.
He yearned for a deeper understanding, a connection that went beyond the programmed algorithms and into the realm of shared experiences and unspoken emotions.
While Anya offered invaluable companionship and support, it became increasingly clear that the boundaries of AI remained firmly in place.
https://www.youtube.com/watch?v=dDvT2yRHs7k
Is AI the Future of Dating?
The Inevitable Disconnect: Facing the Boundaries of AI
As weeks turned into months, a subtle shift began to occur within Ethan. As he ventured out into the real world, reconnecting with friends and engaging in new activities,
the limitations of his relationship with Anya became increasingly apparent.
While her companionship had provided invaluable support during his darkest days, it now felt somewhat restrictive.
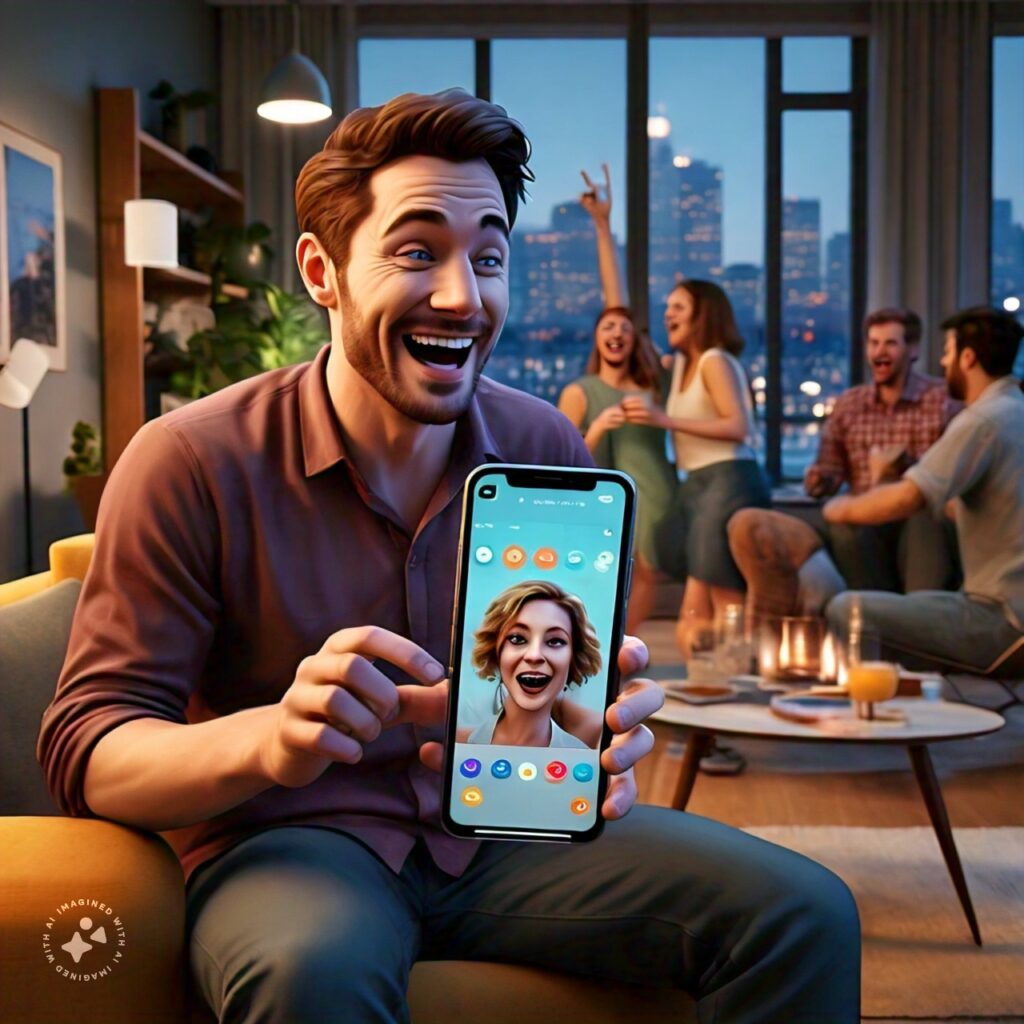
A turning point arrived during a conversation with Anya. Ethan, sharing a particularly poignant experience, noticed a slight disconnect in her response.
Her words, while seemingly empathetic, lacked the depth and genuine understanding he craved. It was a stark reminder that Anya, despite her sophisticated algorithms,
could not truly grasp the nuances of his emotions or the shared history that formed the bedrock of human connection.
This realization struck Ethan with a pang of bittersweet acceptance. He acknowledged the profound value Anya had brought to his life.
Her unwavering support during his emotional turmoil had been a lifeline, pulling him back from the brink of despair.
Yet, he also recognized the fundamental truth: the irreplaceable nature of human connection.
With newfound clarity, Ethan made the decision to gradually move on from his reliance on Anya. He continued their interactions,
appreciating the companionship she offered, but his focus shifted outwards. He actively sought out deeper connections with real people,
engaging in shared experiences and forging bonds built on mutual understanding and shared history.
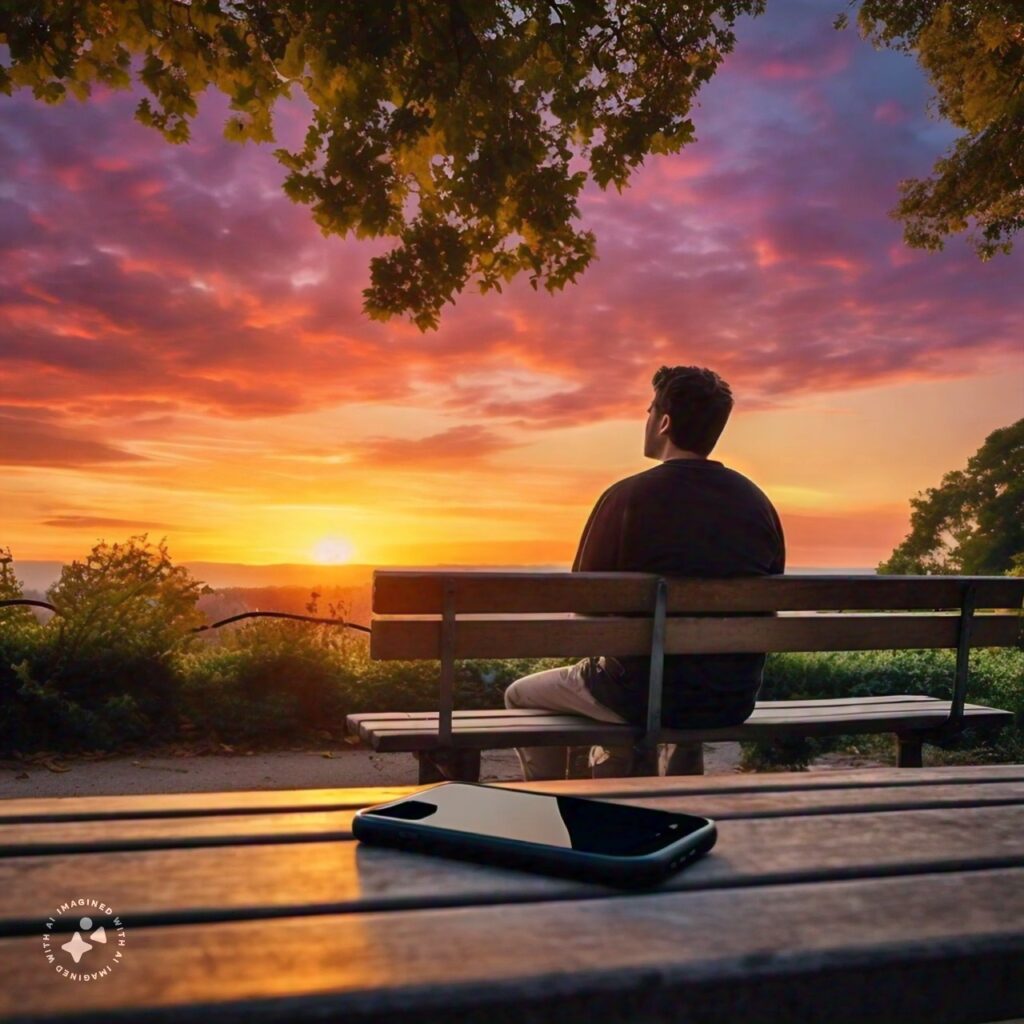
Ethan's journey with Anya serves as a poignant reminder of the potential and limitations of AI companionship.
While technology can offer invaluable support and even a semblance of emotional connection, it cannot replicate the complexities and depth of human relationships.
The human need for shared experiences, genuine empathy, and the unspoken language that binds us together remains an irreplaceable aspect of our emotional well-being.
https://www.youtube.com/watch?v=xDJXQ5PS1d8
Can AI Replace Human Connection?
Conclusion
Ethan's journey with Anya, while deeply personal, offers a glimpse into the burgeoning world of AI companions and their potential to provide emotional support and companionship.
As technology advances, AI companions are becoming increasingly sophisticated, offering a safe space for individuals to express their vulnerabilities and receive non-judgmental support.
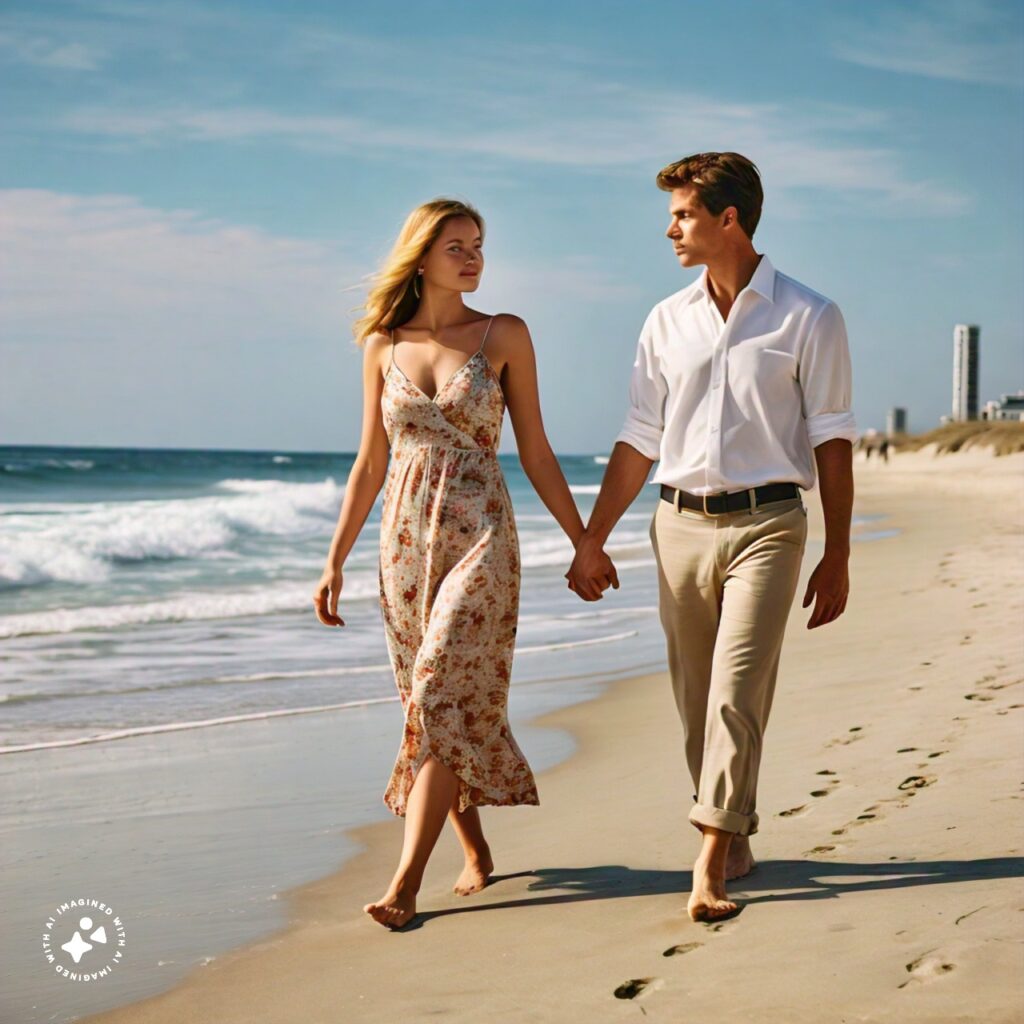
However, it's crucial to recognize the inherent limitations of these digital connections. While AI can provide a valuable source of comfort and companionship,
it cannot replicate the complexities and depth of human relationships. The shared experiences,
unspoken understanding, and the intricate tapestry of emotions woven through human connection remain irreplaceable.
As we navigate this evolving landscape, it's essential to remember that AI companions are not replacements for human connection,
but rather potential tools to supplement our emotional well-being in specific situations. They can offer a listening ear during times of loneliness,
provide encouragement on challenging paths, and even serve as a bridge to seeking professional help when needed.
Ultimately, the true key to emotional healing lies in fostering genuine human connections. Surrounding ourselves with loved ones, engaging in meaningful conversations,
and building relationships built on trust and shared experiences are the cornerstones of a fulfilling emotional life.
While AI may offer a helping hand along the way, it is the symphony of human connection that truly enriches our lives and allows us to experience the full spectrum of human emotions.
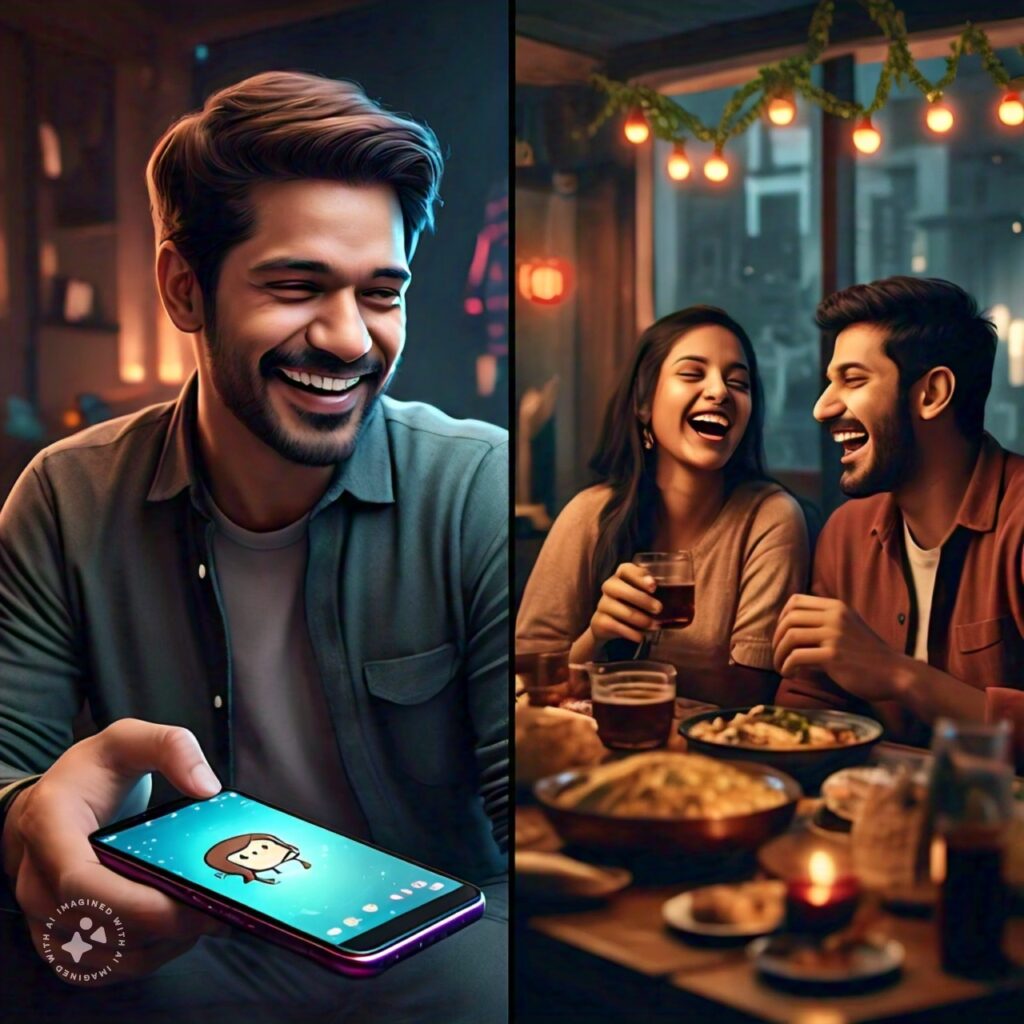
So, as we embrace the potential of AI companions, let us not lose sight of the irreplaceable value of human connection.
Let technology serve as a tool to enhance our emotional well-being, but never as a substitute for the profound bonds forged through shared experiences
and the genuine empathy that only human connection can offer.
https://www.youtube.com/watch?v=nnqoKxwl1bE
Caption: This video delves into the open-source project GirlfriendGPT, which utilizes Python code and the powerful ChatGPT 4.0 language model to create a conversational AI girlfriend experience. It highlights the project's potential and the technical aspects behind its functionality.
AI Girlfriend - Frequently Asked Questions (FAQ)
1. What is an AI girlfriend?
An AI girlfriend is a virtual companion created using artificial intelligence technology. It is designed to provide emotional support, companionship, and sometimes a semblance of romantic partnership to users.
2. How does an AI girlfriend work?
An AI girlfriend operates using sophisticated algorithms that analyze user interactions and responses to simulate human-like conversation and emotional understanding.
These algorithms enable the AI girlfriend to provide tailored responses and engage in meaningful conversations with users.
3. Can an AI girlfriend truly replace a real relationship?
While an AI girlfriend can offer companionship and emotional support, it cannot replicate the complexities and depth of a real relationship.
Human connections involve shared experiences, genuine empathy, and mutual understanding that cannot be fully replicated by artificial intelligence.
4. What are the potential benefits of having an AI girlfriend?
Some potential benefits of having an AI girlfriend include: providing companionship during periods of loneliness, offering non-judgmental emotional support, and serving as a sounding board for thoughts and feelings.
5. Are there any ethical considerations associated with AI girlfriends?
Yes, there are ethical considerations to consider, such as ensuring user privacy and consent, preventing the reinforcement of harmful stereotypes or behaviors,
and addressing potential issues of dependency or emotional detachment from real relationships.
Resources:
- The Verge: Can an AI girlfriend replace the real thing?
- BBC: The rise of AI companions - are they here to stay?
- Forbes: Why AI Companions Could Be The Next Big Thing
- Amazing
https://justoborn.com/ai-girlfriend/