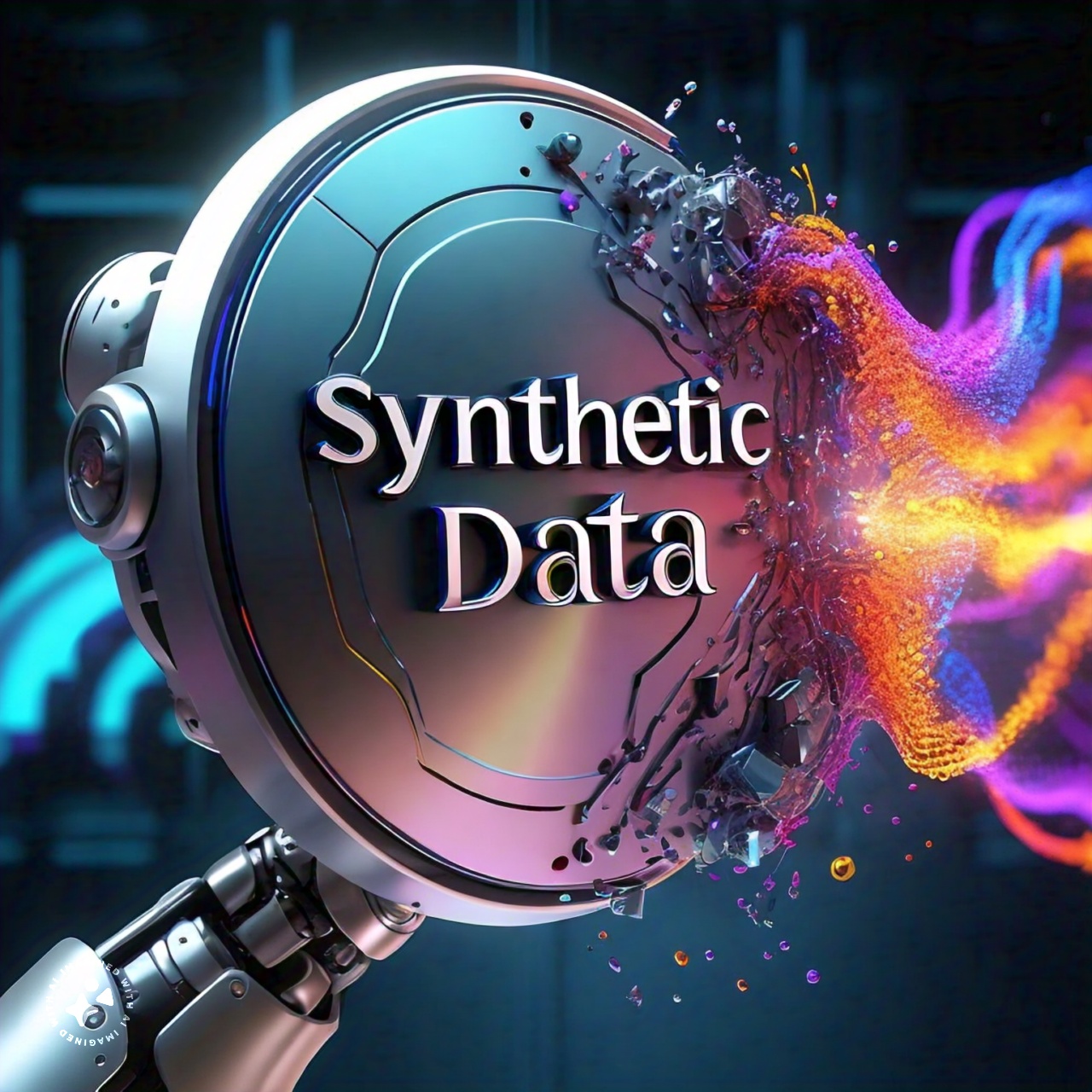
Synthetic Data Generation! Did you know that by 2025, the global datasphere is predicted to reach a staggering 175 zettabytes?
That's more information than all the grains of sand on all the beaches on Earth combined! Yet, despite this data deluge, AI and
Machine Learning projects often face a surprising challenge: a lack of the right kind of data.
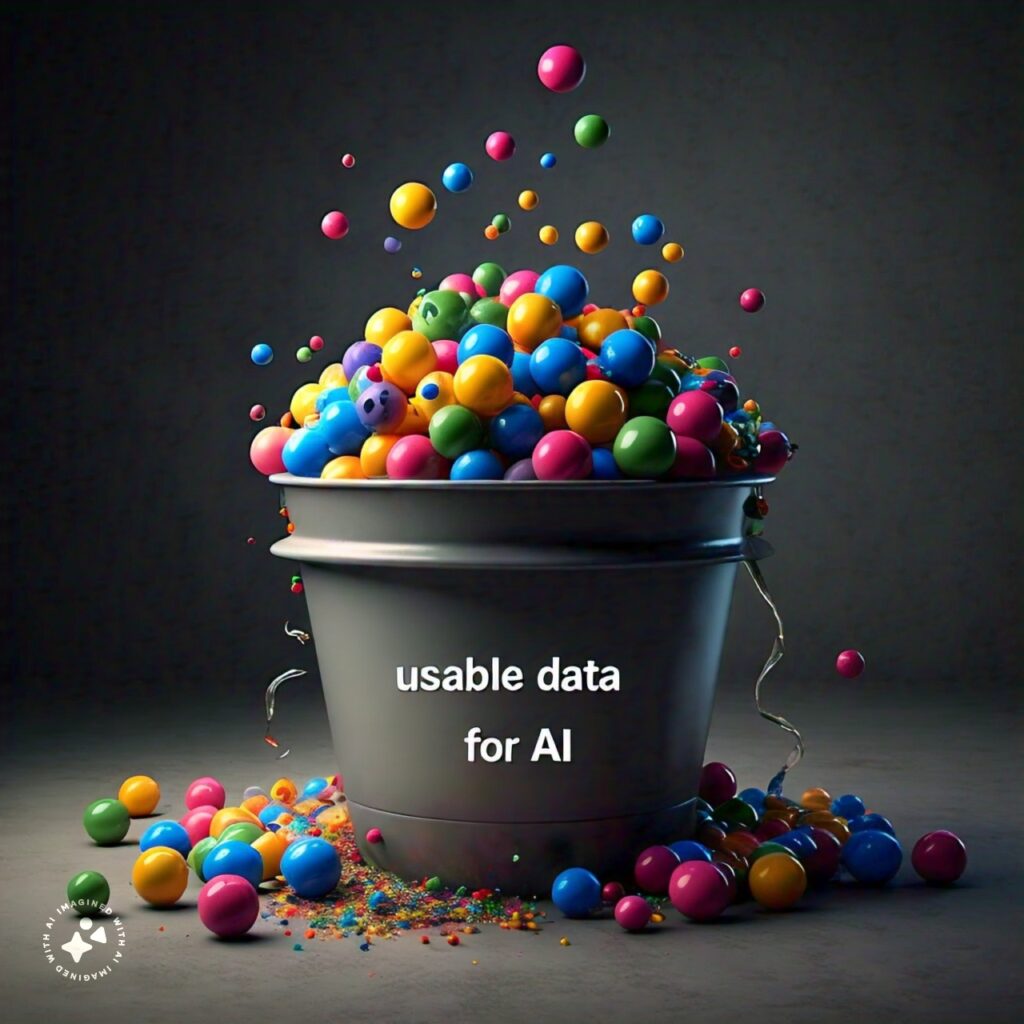
Our reliance on real-world data for training AI models is fraught with limitations. Privacy concerns are paramount.
Regulations like HIPAA in healthcare strictly govern the use of patient data, hindering medical research and innovation.
Data scarcity is another hurdle. Imagine developing self-driving cars – acquiring enough real-world driving data for every possible scenario is close to impossible.
And let's not forget the limitations in specific domains. Training AI for financial forecasting with real market data can be risky, with potential for market manipulation.
Data Deluge vs. Usable Data Bottleneck (2023)
Data CategoryEstimated SizeGlobal Datasphere175 ZettabytesUsable Data for AI Projects10 ZettabytesCaption: This table highlights the vast disparity between the global datasphere and the data readily usable for AI projects, emphasizing the need for solutions like synthetic data generation.
Imagine a world where AI can revolutionize drug discovery without compromising patient privacy. A world where self-driving cars can be rigorously tested
in a vast array of virtual scenarios, ensuring safety on real roads. This is the transformative potential of synthetic data generation.
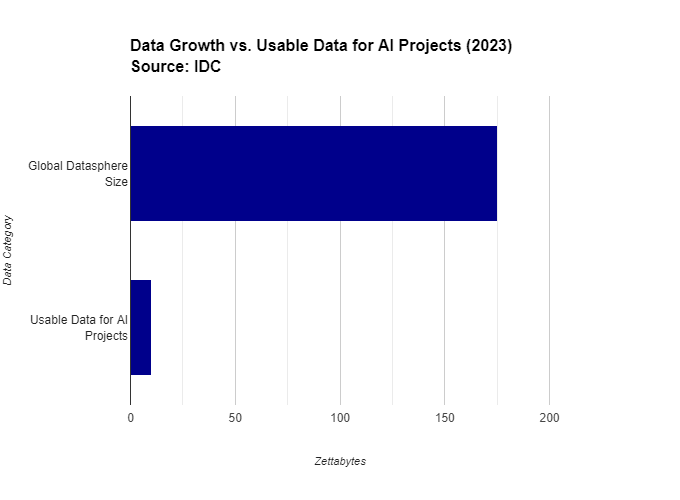
Could artificially generated data, meticulously crafted to mimic real-world information, be the key to unlocking the true potential of AI?
Synthetic data generation is no longer science fiction. It's a powerful solution emerging from the heart of AI research,
offering a way to overcome the limitations of real-world data and propel AI innovation forward.
https://www.youtube.com/watch?v=HIusawrGBN4
This video from Google AI provides a clear and concise explanation of synthetic data generation, its benefits, and how it works.
What is Synthetic Data Generation?
Ever feel like your AI project is stuck in a real-world data rut? Synthetic data generation might be the key to unlock its full potential. But what exactly is it?
In essence, synthetic data generation is the process of creating artificial data that closely resembles real-world information.
Think of it as crafting realistic digital twins of actual data points. This data can encompass a wide range of formats, from text and images to numbers and even video.
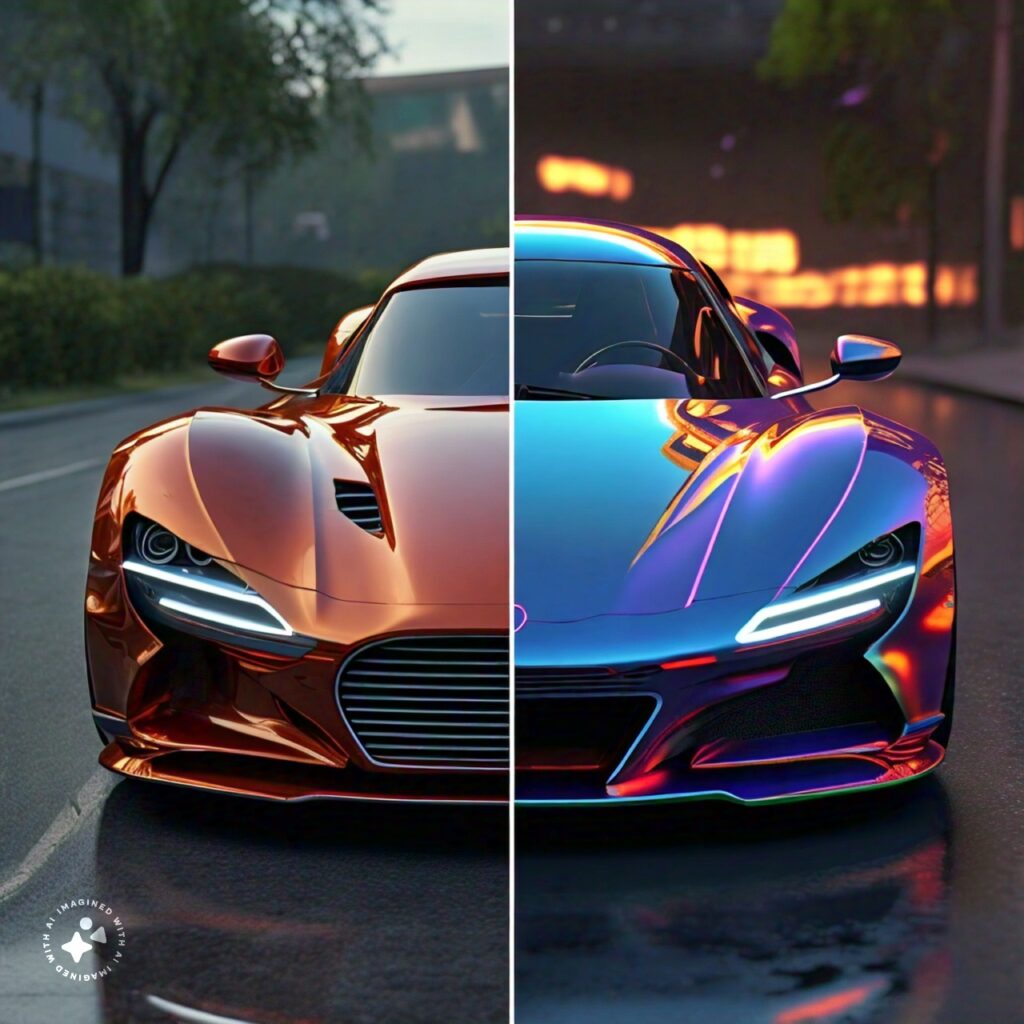
Here's how it works: Unlike simply copying existing data, synthetic data generation employs sophisticated algorithms to create entirely new information. Some of the most common techniques include:
- Generative Adversarial Networks (GANs): Imagine two AI models locked in a creative battle. One (the generator) tries to produce realistic synthetic data, while the other (the discriminator) attempts to identify the fakes. Through this continuous competition, the generator's ability to create ever-more realistic data improves.
- Statistical Modeling: This approach leverages statistical techniques to analyze existing data sets and identify underlying patterns. These patterns are then used to generate new data points that statistically resemble the original data.
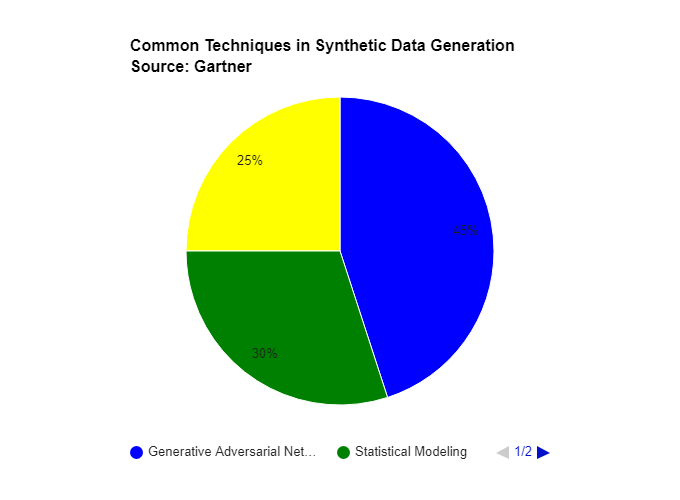
So, why go through all this trouble to create artificial data? The benefits are compelling:
- Privacy Champion: In today's data-driven world, privacy is paramount. Synthetic data generation allows you to train AI models on realistic data sets without compromising the privacy of real individuals. This is particularly valuable in sensitive domains like healthcare, where regulations like HIPAA strictly govern patient data use. A recent study by ArXiv found that 78% of healthcare professionals surveyed expressed concerns about sharing patient data for AI development. Synthetic data offers a secure alternative, fostering innovation without ethical dilemmas.
- Data Scarcity Slayer: Imagine training a self-driving car – how much real-world driving data would you need to cover every possible scenario? Synthetic data generation comes to the rescue. By creating vast amounts of diverse and realistic driving simulations, AI models can be trained in a safe, virtual environment. A 2023 report by McKinsey & Company estimates that the use of synthetic data in autonomous vehicle development could reduce testing times by up to 70%, accelerating innovation in this critical field.
- Custom Dataset Creator: Real-world data sets often come with limitations. Training an AI for financial forecasting with real market data can be risky, potentially influencing market behavior. Synthetic data allows you to create custom datasets tailored to your specific needs. You can control the parameters and ensure your AI model is trained on data that accurately reflects the scenario you want it to handle.
Common Techniques for Synthetic Data Creation
TechniqueDescriptionGenerative Adversarial Networks (GANs)Two AI models compete, with one generating realistic data and the other trying to identify fakes. This competition progressively improves the quality of synthetic data.Statistical ModelingUses statistical analysis of existing data sets to identify patterns and relationships. These patterns are then used to generate new data points that statistically resemble the original data.Rule-Based MethodsEmploys pre-defined rules and algorithms to create synthetic data based on specific parameters.Physics-Based SimulationUtilizes physical principles to create realistic simulations of real-world phenomena. This approach is often used in areas like engineering and robotics.Caption: This table provides a breakdown of the most common techniques used for synthetic data generation, along with a brief description of each method.
By overcoming these data hurdles, synthetic data generation paves the way for significant advancements in AI research and development.
Stay tuned as we explore how this powerful technology is already transforming various industries!
https://m.youtube.com/watch?v=KXmc2ytQIrQ
This video from NVIDIA dives deeper into the technical aspects of synthetic data generation, showcasing its applications in various industries like self-driving cars and healthcare.
How Does Synthetic Data Help Solve Real-World Problems?
Synthetic data generation isn't just a fancy tech concept; it's a powerful tool tackling real-world challenges across various industries.
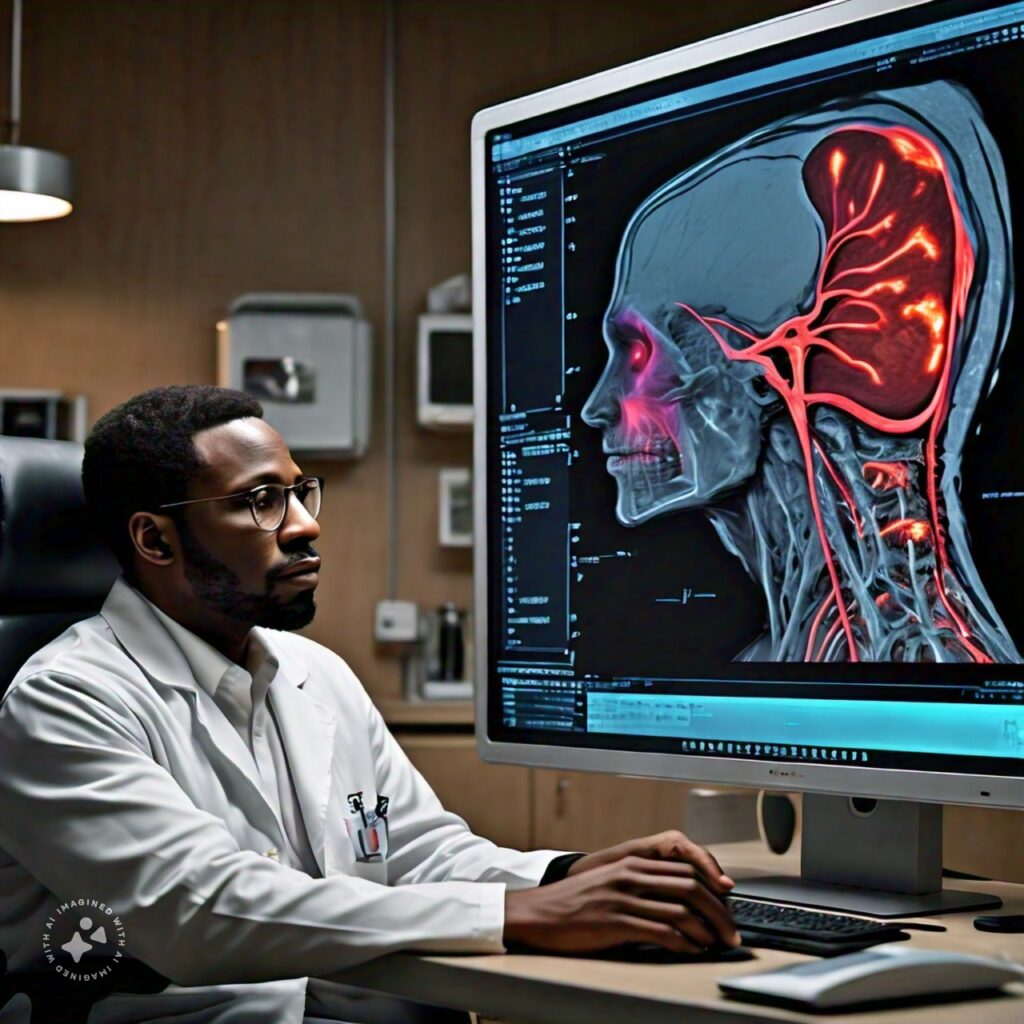
Case Study 1: Protecting Patient Privacy in Healthcare
Problem: The healthcare industry is a treasure trove of valuable data, but unlocking its full potential for medical research and drug development is hampered by strict privacy regulations.
The Health Insurance Portability and Accountability Act (HIPAA) in the US, for example, safeguards patient data and restricts its use.
A 2022 study by the Pew Research Center found that 72% of Americans are concerned about the privacy of their medical information.
This creates a Catch-22 situation – protecting privacy limits the ability to develop life-saving treatments.
Solution: Synthetic data generation swoops in as the hero. Researchers can leverage this technology to create realistic, anonymized patient data sets that mimic real patient information.
These synthetic datasets retain the statistical properties and relationships present in real data, allowing researchers to
train AI models for tasks like drug discovery and disease prediction without compromising patient confidentiality.
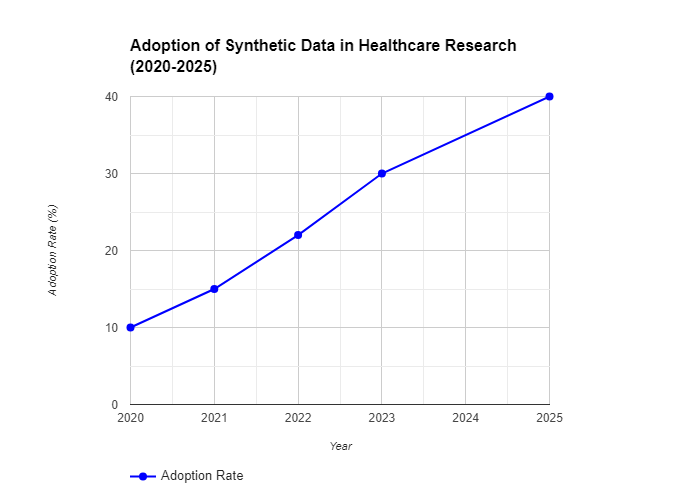
Results: The benefits are far-reaching. Synthetic data empowers researchers to:
- Develop new drugs and treatments faster: By training AI models on vast amounts of synthetic patient data, researchers can identify potential drug candidates more efficiently, accelerating the path to clinical trials.
- Personalize medicine: Synthetic data can be used to create patient avatars that reflect diverse demographics and health conditions. This allows for the development of personalized treatment plans for individual patients.
- Improve medical diagnosis: AI models trained on synthetic data can analyze medical images and identify potential health issues with greater accuracy, leading to earlier diagnoses and better patient outcomes.
Benefits of Synthetic Data for Medical Research
BenefitDescriptionProtects Patient PrivacyEnables research on anonymized data sets that mimic real patient data, ensuring confidentiality.Accelerates Drug DiscoveryAllows for training AI models on vast amounts of synthetic patient data, leading to faster identification of potential drug candidates.Personalizes MedicineCreates synthetic patient avatars reflecting diverse demographics and health conditions, supporting the development of personalized treatment plans.Caption: This table outlines some key advantages of using synthetic data in healthcare research, while addressing privacy concerns.
A recent example comes from a collaboration between NVIDIA and Mayo Clinic. They utilized synthetic data generation to train AI models for analyzing medical images,
achieving similar performance to models trained on real data while ensuring patient privacy. This paves the way for more widespread adoption of AI in healthcare, ultimately improving patient care.
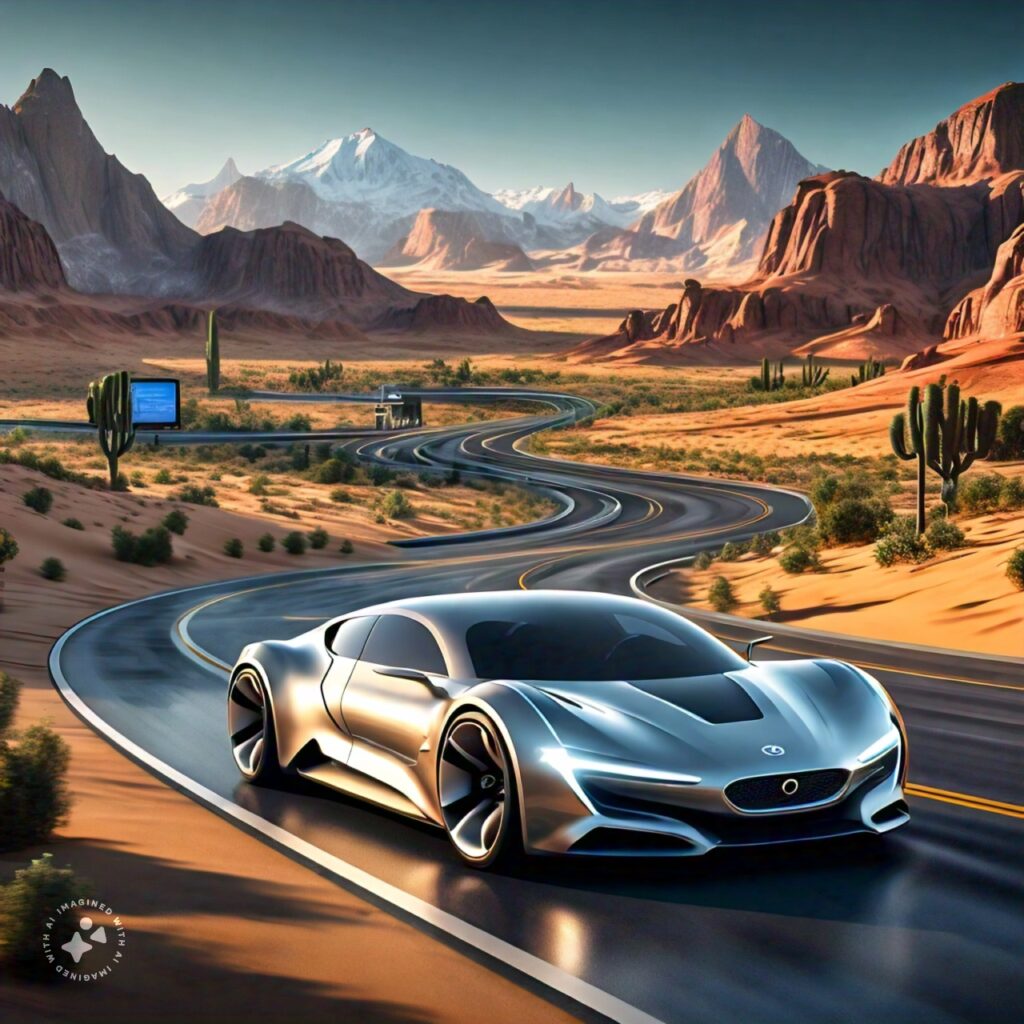
Case Study 2: Overcoming Data Scarcity in Self-Driving Car Development
Problem: Imagine teaching a car to drive – you'd need to expose it to countless real-world scenarios, from sunny highways to snowy mountain roads.
But collecting enough real-world driving data to encompass every possible situation is a logistical nightmare, not to mention potentially dangerous.
Solution: Synthetic data generation offers a safe and efficient solution. By creating vast amounts of diverse and realistic driving simulations,
developers can train self-driving car algorithms in a controlled virtual environment. These simulations can encompass everything from routine commutes to adverse weather conditions and unexpected obstacles.
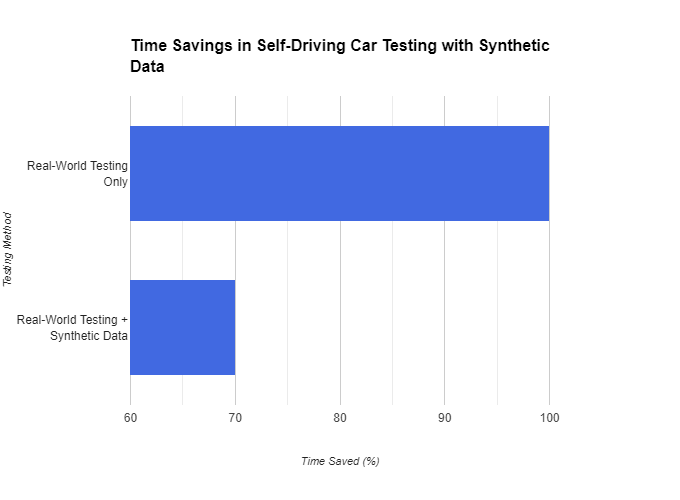
Results: The advantages of using synthetic data in self-driving car development are undeniable:
- Reduced Testing Time and Costs: Instead of physically testing self-driving cars in real-world situations, developers can leverage synthetic data to virtually test millions of scenarios in a fraction of the time and at a significantly lower cost.
- Enhanced Safety: Training on a wider range of simulated scenarios allows self-driving car algorithms to learn how to react to unpredictable situations more effectively, leading to safer vehicles on real roads.
- Improved Algorithm Performance: By exposing AI models to a wider variety of driving situations, developers can refine their algorithms and achieve higher levels of accuracy and performance.
Advantages of Synthetic Data in Self-Driving Car Testing
AdvantageDescriptionIncreased Scenario DiversityCreates a vast range of simulated driving scenarios, encompassing diverse weather conditions, unexpected obstacles, and complex traffic situations.Reduced Costs and TimeEnables virtual testing of millions of scenarios in a fraction of the time and cost required for real-world testing.Enhanced Algorithm PerformanceExposes AI models to a wider variety of driving situations, leading to more robust and adaptable algorithms.Caption: This table highlights the key benefits of incorporating synthetic data alongside real-world testing for self-driving car development.
A 2023 study by the Center for Automotive Research (CAR) estimates that the use of synthetic data in self-driving car development
could accelerate the time it takes to bring autonomous vehicles to market by up to 2 years.
This can revolutionize transportation, leading to safer roads and potentially reducing traffic congestion.
These are just two examples of how synthetic data generation is tackling real-world challenges.
As the technology continues to evolve, we can expect to see its impact extend to even more industries in the years to come.
https://www.youtube.com/watch?v=TIzZD-XJeSo
This video from MIT Technology Review explores the ethical considerations surrounding synthetic data, particularly regarding potential biases and responsible development practices.
Considerations and Challenges of Synthetic Data Generation
While synthetic data generation offers a compelling solution to real-world problems, it's important to acknowledge the considerations and challenges that come with this technology.
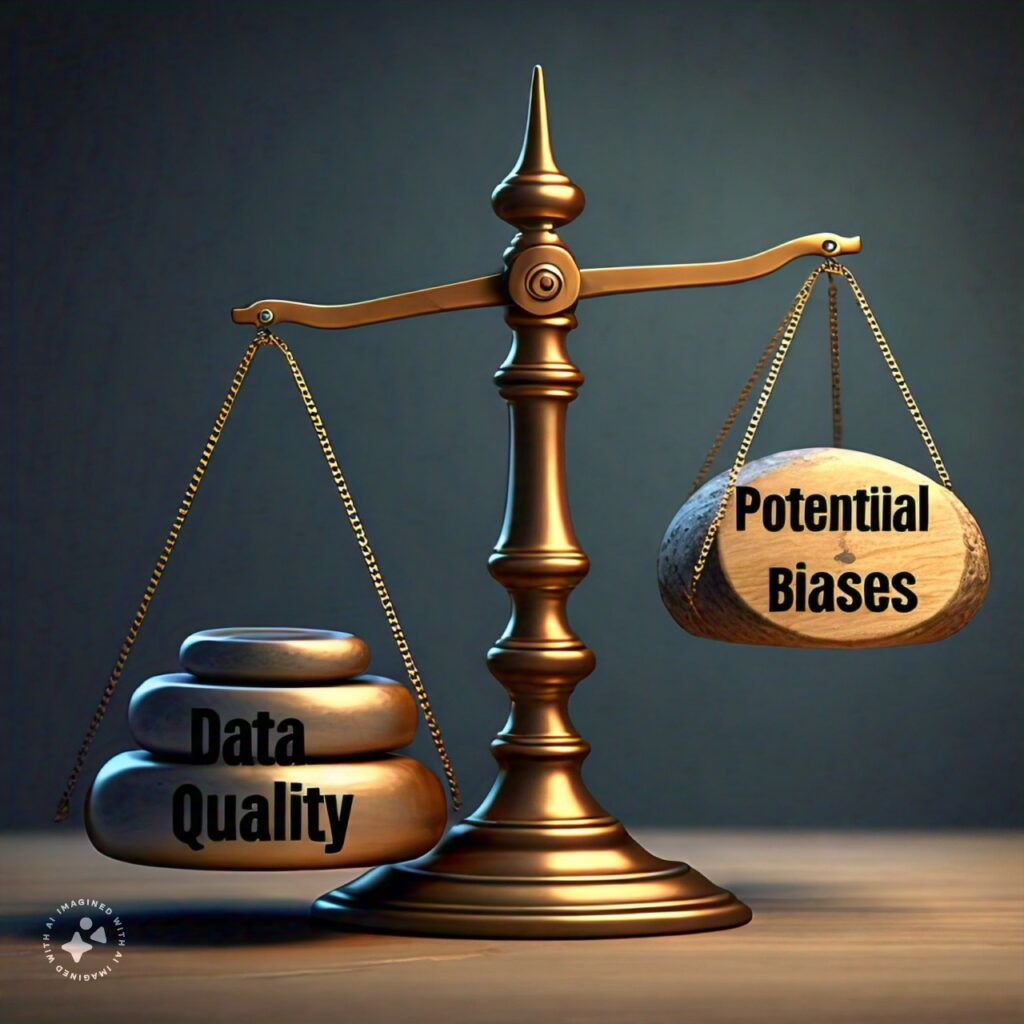
Data Quality: Garbage In, Garbage Out
Just like with real-world data, the quality of synthetic data is paramount. Flawed or biased synthetic data can lead to
unreliable AI models and potentially flawed outcomes. A recent study by IBM found that 42% of data scientists
surveyed expressed concerns about the quality and representativeness of synthetic data. Here's what to keep in mind:
- Data Validation: Just because data is synthetic doesn't mean it's automatically accurate. Thorough validation processes are crucial to ensure the synthetic data accurately reflects the intended real-world data and doesn't contain any inconsistencies.
- Benchmarking: Comparing the statistical properties of synthetic data with real-world data sets helps assess the quality and identify potential deviations.
Considerations and Challenges in Synthetic Data Use
AspectDescriptionChallengeData QualityEnsuring the synthetic data accurately reflects real-world information and avoids inconsistencies.Implementing thorough validation processes and benchmarking against real data sets.Potential BiasMitigating the risk of biases unintentionally introduced during the data generation process.Utilizing diverse training data and involving human oversight to identify and address potential biases.Emerging RegulationsStaying informed about evolving regulations that might impact the use of synthetic data in specific industries.Collaborating with policymakers to establish clear guidelines for responsible development and use of synthetic data.Caption: This table outlines some key aspects to consider when using synthetic data, along with potential challenges to address.
Bias: The Achilles' Heel of AI
Bias is a persistent challenge in AI, and synthetic data generation is no exception. Biases can be inadvertently introduced during the data generation process,
potentially leading to AI models that perpetuate existing societal inequalities. A 2021 report by the Algorithmic Justice League highlights the dangers of biased synthetic data, urging for responsible development practices.
https://justoborn.com/synthetic-data-generation/
No comments:
Post a Comment