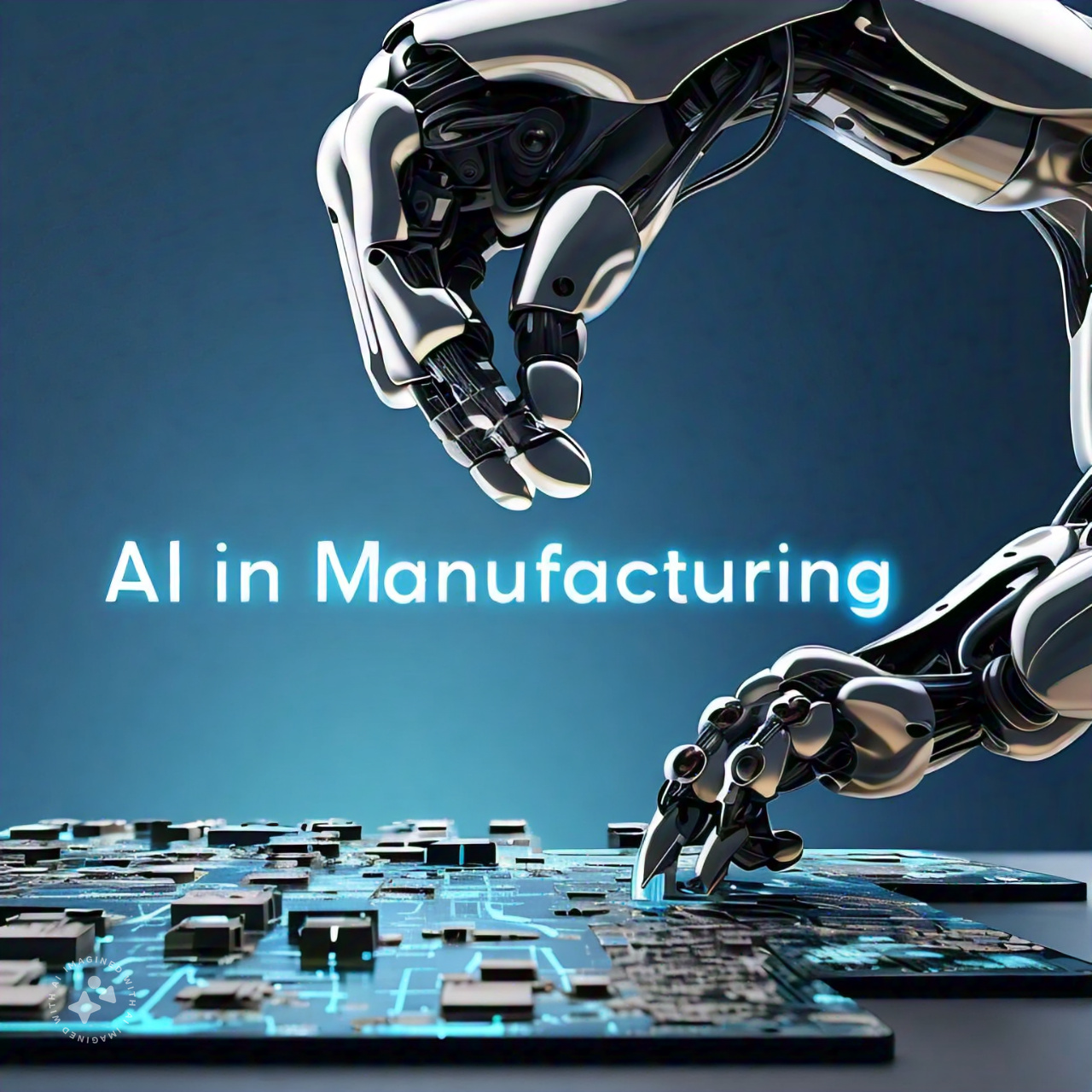
AI in Manufacturing! Imagine a world where machines not only work tirelessly but also think, learn, and adapt.
This isn't science fiction—it's the reality of AI in manufacturing, the cornerstone of Industry 4.0. As we stand on the brink of a new industrial revolution,
AI is transforming factories into smart, efficient powerhouses of production.
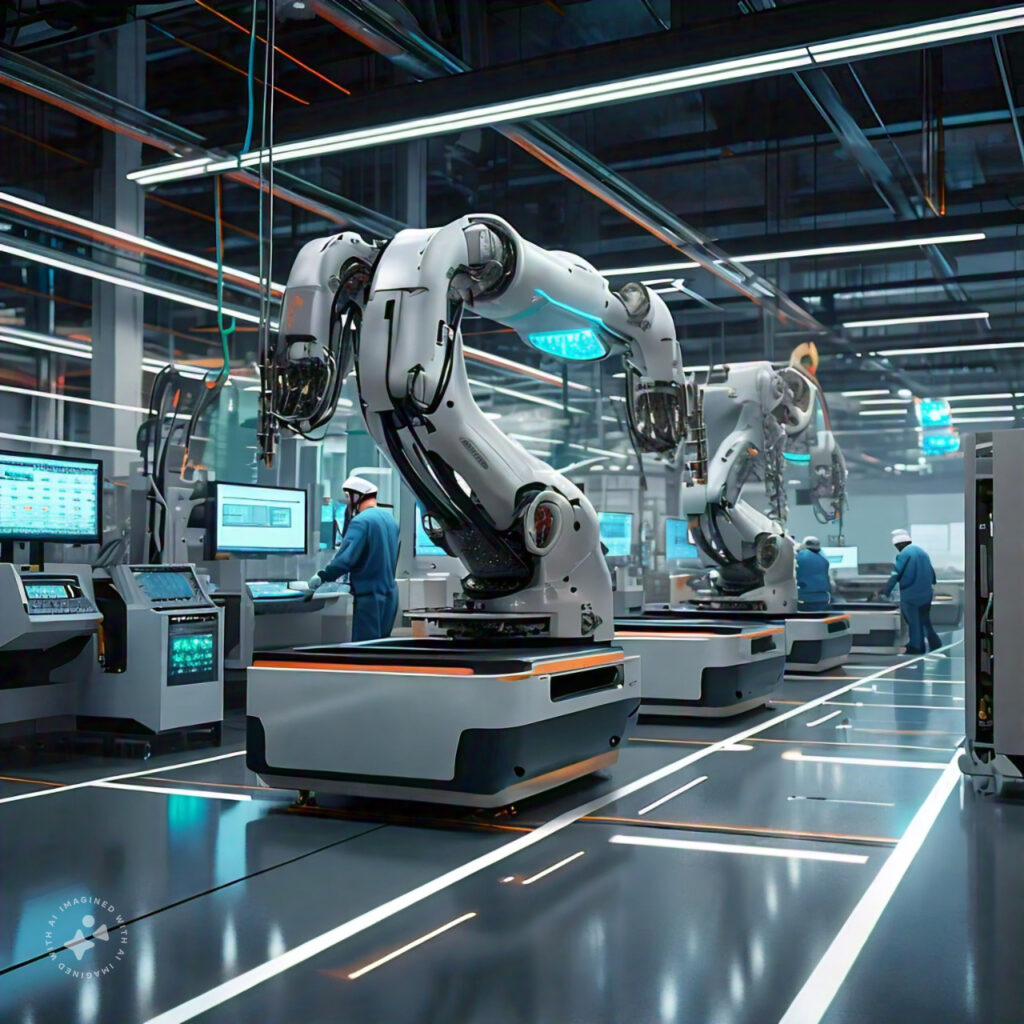
Did you know that AI-powered predictive maintenance can reduce machine downtime by up to 50% and increase machine life by 20-40%?
McKinsey & Company (2021) This game-changing capability is just one of the many ways AI is revolutionizing the manufacturing landscape,
promising to boost global GDP by $15.7 trillion by 2030 PwC (2023).
As AI continues to evolve, how will the role of human workers in manufacturing change, and what new skills will be essential for the factory workers of tomorrow?
Picture Sarah, a quality control inspector at a bustling automotive plant. For years, she meticulously examined each component,
her trained eye catching defects that could compromise safety. Today, Sarah works alongside an AI system that can detect microscopic flaws in milliseconds.
Instead of feeling replaced, Sarah feels empowered. She now focuses on interpreting AI insights, fine-tuning the system, and tackling complex issues that require human intuition.
Sarah's story is a microcosm of the AI revolution in manufacturing—where human expertise and artificial intelligence combine to create unprecedented efficiency and innovation.
In the fast-paced world of modern manufacturing, Artificial Intelligence (AI) has emerged as a game-changing force,
reshaping the industry's landscape and ushering in the era of Industry 4.0. This technological revolution is not just about robots on assembly lines;
it's about creating smart, interconnected systems that can think, learn, and adapt in real-time.
AI in manufacturing encompasses a wide range of technologies, from machine learning algorithms that predict equipment failures to computer vision systems that
detect microscopic defects invisible to the human eye. These AI-powered solutions are transforming every aspect of the production process,
from design and planning to quality control and supply chain management.
AI in Manufacturing: Key Statistics
Metric
Value
Year
Global AI in Manufacturing Market Size
$2.3 billion
2022
Projected Market Size
$16.3 billion
2027
CAGR
47.9%
2022-2027
Companies reporting cost savings
79%
2022
The importance of AI in Industry 4.0 cannot be overstated. As factories become increasingly digitized and
interconnected, AI serves as the brain that processes vast amounts of data, derives meaningful insights, and makes split-second decisions.
This capability is crucial in today's competitive global market, where efficiency, quality, and adaptability can make or break a company's success.
Recent statistics underscore the growing significance of AI in manufacturing:
- The global AI in manufacturing market is projected to reach $16.7 billion by 2026, growing at a CAGR of 57.2% from 2020 MarketsandMarkets (2023).
- 87% of manufacturers believe AI will be essential for their survival over the next five years Deloitte (2022).
- AI-enabled predictive maintenance can increase equipment availability by up to 20% and reduce maintenance costs by up to 40% McKinsey & Company (2021).
As we delve deeper into the world of AI in manufacturing, we'll explore how this technology is not just changing what we make, but how we make it.
From smart factories that optimize their own operations to AI-assisted design processes that push the boundaries of innovation,
the future of manufacturing is being written in lines of code, and the possibilities are limitless.
The Building Blocks of AI in Manufacturing
The foundation of AI in manufacturing rests on three key technologies: Machine Learning, Computer Vision, and Natural Language Processing.
These building blocks work together to create intelligent systems that can revolutionize production processes, quality control, and decision-making in factories.
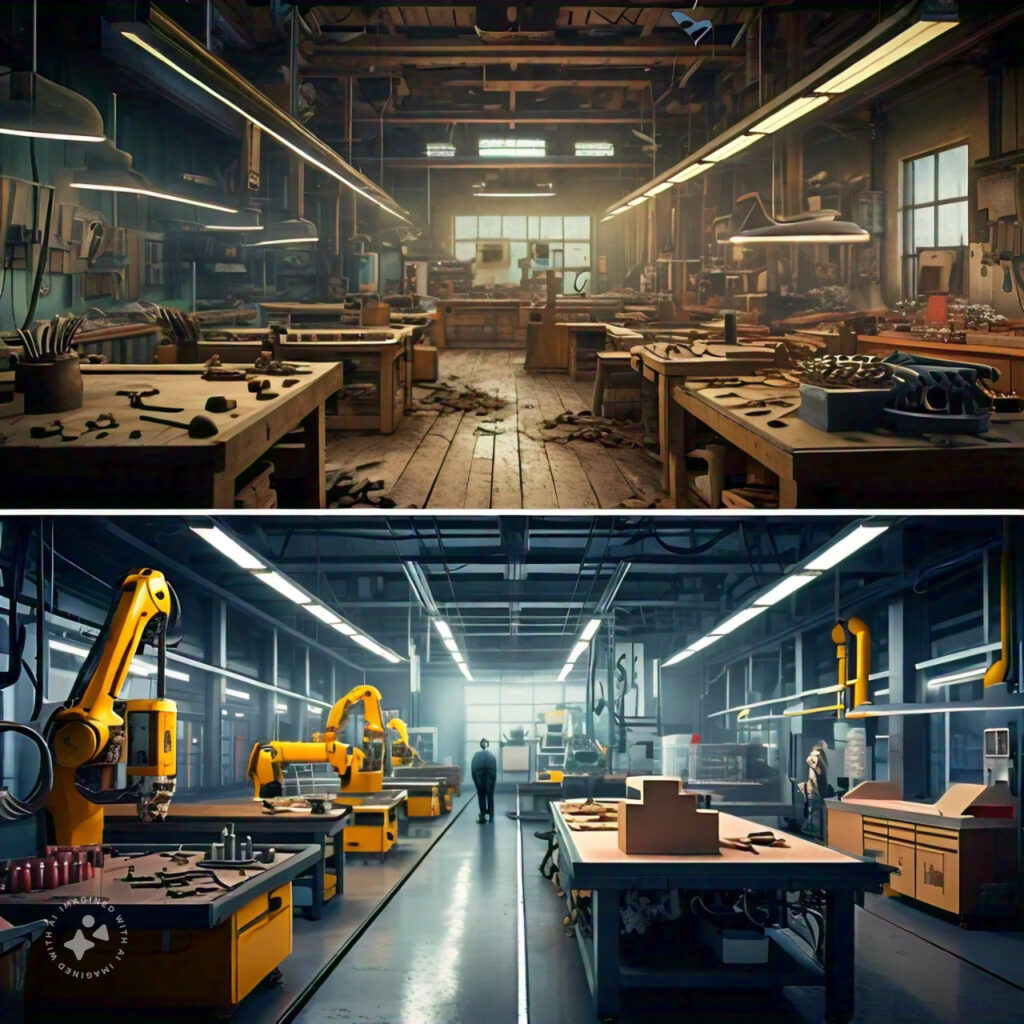
A. Machine Learning
Machine Learning (ML) is the cornerstone of AI in manufacturing. It enables systems to learn from data and improve their performance over time without being explicitly programmed.
In manufacturing, ML algorithms analyze vast amounts of production data to identify patterns, predict outcomes, and optimize processes.
A recent study by Deloitte (2023) found that 87% of manufacturers believe ML will be essential for their survival over the next five years.
One of the most impactful applications of ML in manufacturing is predictive maintenance. By analyzing sensor data from equipment,
ML algorithms can predict when a machine is likely to fail, allowing for proactive maintenance and reducing costly downtime.
For example, a leading automotive manufacturer implemented an ML-based predictive maintenance system that reduced unplanned downtime by 20% and maintenance costs by 15%.
This showcases the tangible benefits ML can bring to the manufacturing floor.
AI in Manufacturing: Key Concepts
AI-Powered Robotics
Collaborative robots (cobots) increasing efficiency in manufacturing processes.
Predictive Maintenance
AI predicts equipment failures before they happen, reducing downtime.
Quality Control
Real-time defect detection for higher quality products.
AI Applications
Diverse use cases of AI in various manufacturing processes.
Enhanced Product Quality
AI ensures consistent and superior product quality.
Cost Savings
AI optimizes processes, leading to significant cost reductions.
Improved Safety
AI enhances workplace safety through predictive analytics and automation.
Data-Driven Insights
AI leverages big data for smarter decision-making in manufacturing.
B. Computer Vision
Computer Vision (CV) gives machines the ability to "see" and interpret visual information, much like the human eye and brain.
In manufacturing, CV is transforming quality control, inventory management, and worker safety.
According to McKinsey & Company (2022), CV can improve defect detection rates by up to 90% compared to manual inspection.
This level of accuracy is crucial in industries where even minor defects can lead to significant issues, such as in electronics or aerospace manufacturing.
A real-world example of CV's impact comes from a semiconductor manufacturer that implemented a CV system for wafer inspection.
The system increased defect detection accuracy by 95% while reducing inspection time by 70%, significantly improving both quality and efficiency.
Key Takeaways: AI in Manufacturing
Efficiency Boost
AI significantly improves manufacturing efficiency through process optimization and automation.
Up to 20% increase in productivity reported by early adopters.
Learn More
Quality Control
AI-powered visual inspection systems dramatically improve product quality and reduce defects.
Defect detection rates improved by up to 90% in some cases.
Explore AI in QC
Predictive Maintenance
AI predicts equipment failures before they occur, reducing downtime and maintenance costs.
Unplanned downtime reduced by up to 50% in some industries.
Discover Predictive AI
Supply Chain Optimization
AI enhances supply chain management through improved forecasting and logistics optimization.
Inventory costs reduced by up to 30% with AI-driven supply chain management.
Optimize Your Supply Chain
C. Natural Language Processing
Natural Language Processing (NLP) enables machines to understand, interpret, and generate human language.
In manufacturing, NLP is enhancing communication between humans and machines, improving documentation processes, and facilitating more intuitive interfaces for complex systems.
Gartner (2023) predicts that by 2025, 50% of manufacturing execution systems will incorporate NLP capabilities, enabling more natural interactions between workers and machines.
One innovative application of NLP in manufacturing is in maintenance documentation. A global aerospace manufacturer implemented an NLP system that
automatically generates maintenance reports from technician voice notes. This system reduced report creation time by 60% and improved the accuracy and consistency of documentation.
These building blocks of AI - Machine Learning, Computer Vision, and Natural Language Processing - are not just theoretical concepts but
practical tools that are already reshaping the manufacturing landscape. As these technologies continue to evolve and
integrate, they promise to unlock new levels of efficiency, quality, and innovation in the factories of the future.
Key Applications of AI in Factories
The integration of AI in manufacturing has revolutionized factory operations, bringing unprecedented levels of efficiency, precision, and productivity.
Let's explore three key applications that are transforming the industry:
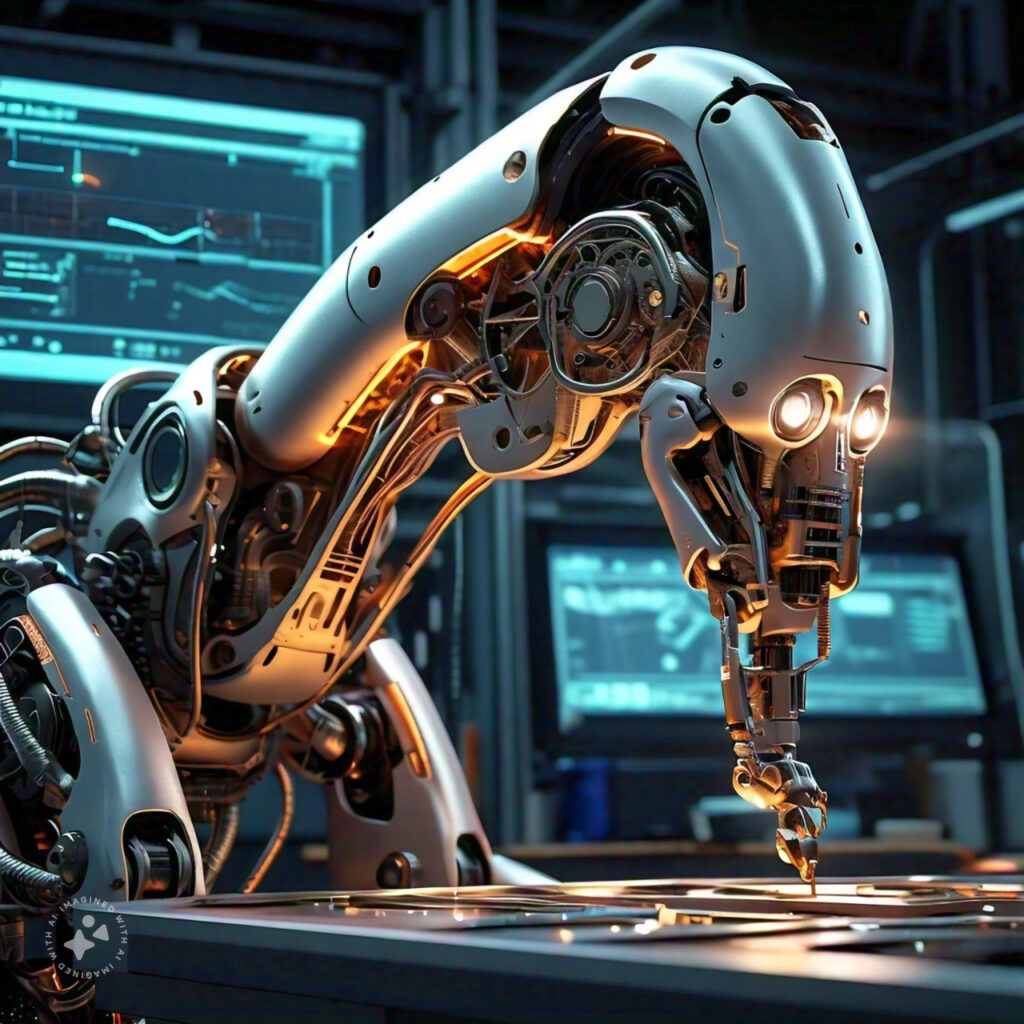
A. AI-Powered Robotics
- Collaborative robots (cobots)
Cobots represent a significant leap in human-robot collaboration. Unlike traditional industrial robots, cobots are designed to work alongside human workers,
enhancing productivity and safety. These AI-driven machines can adapt to their environment and learn new tasks quickly.
According to a recent report by the International Federation of Robotics (2023), cobot installations increased by 22% in 2022, with over 41,000 units deployed globally.
This surge in adoption is driven by their versatility and ability to augment human capabilities rather than replace workers.
For instance, BMW has implemented cobots in their assembly lines to assist workers with ergonomically challenging tasks.
These cobots, equipped with advanced sensors and AI algorithms, can handle delicate components and work in close proximity to humans without safety concerns.
- Autonomous mobile robots (AMRs)
AMRs are revolutionizing material handling and logistics within factories. These self-navigating robots use AI to plan optimal routes, avoid obstacles, and adapt to changing factory layouts.
ABI Research (2023) predicts that the global market for AMRs will reach $100 billion by 2030, highlighting their growing importance in manufacturing.
AMRs are particularly valuable in large-scale operations where efficiency in material movement is crucial.
Amazon's fulfillment centers showcase the power of AMRs. Their latest robot, Proteus, uses advanced AI to safely navigate around human workers,
optimizing warehouse operations and significantly reducing order processing times.
How AI Learning Works: A Timeline
Data Collection
AI systems gather large amounts of data from various sources to learn from.
Data Preprocessing
Raw data is cleaned, normalized, and prepared for analysis.
Feature Extraction
Important features are identified and extracted from the preprocessed data.
Model Selection
An appropriate AI model is chosen based on the problem and data type.
Training
The AI model learns patterns from the training data through iterative processes.
Validation
The model's performance is evaluated using a separate validation dataset.
Fine-tuning
The model is adjusted and optimized based on validation results.
Deployment
The trained and validated AI model is deployed for real-world use.
B. Predictive Maintenance
- How AI predicts equipment failures
Predictive maintenance powered by AI is transforming how factories manage their equipment. By analyzing vast amounts of sensor data,
AI algorithms can detect subtle patterns that indicate potential failures, often weeks or months in advance.
These systems use machine learning models trained on historical data to recognize anomalies in equipment behavior.
They consider factors such as vibration, temperature, and power consumption to predict when a machine is likely to fail.
- Case study: Siemens' success with predictive maintenance
Siemens (2022) has implemented an AI-driven predictive maintenance system across its global operations. By analyzing data from millions of sensors, the system has:
- Reduced unplanned downtime by up to 50%
- Increased overall equipment effectiveness by 20%
- Saved millions in maintenance costs annually
The system not only predicts failures but also recommends optimal maintenance schedules, ensuring that repairs are conducted at the most cost-effective times.
Key Features of AI in Manufacturing
Predictive Maintenance
AI algorithms analyze sensor data to predict when equipment is likely to fail, allowing for proactive maintenance.
Benefit: Reduces unplanned downtime by up to 50%
Example: GE's Predix platform for industrial IoT and predictive maintenance
Quality Control
AI-powered computer vision systems inspect products for defects with greater accuracy and speed than human inspectors.
Benefit: Improves defect detection rates by up to 90%
Example: BMW's AI-based visual inspection system for car body parts
Process Optimization
AI algorithms analyze production data to optimize manufacturing processes, improving efficiency and reducing waste.
Benefit: Increases overall equipment effectiveness by up to 20%
Example: Siemens' AI-powered optimization of gas turbine production
Collaborative Robots
AI-powered robots work alongside human workers, enhancing productivity and safety in manufacturing environments.
https://justoborn.com/ai-in-manufacturing/
No comments:
Post a Comment