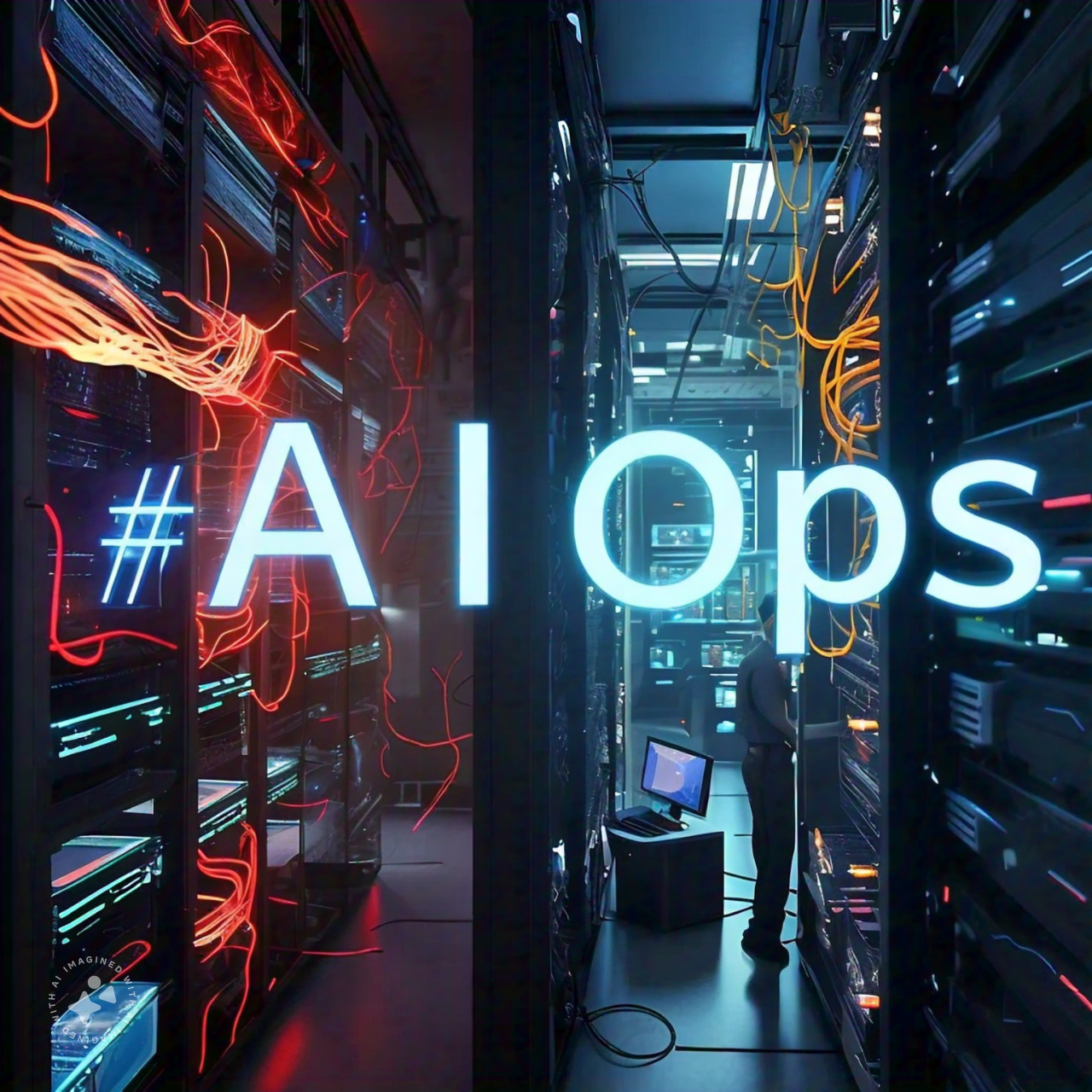
AI Ops! Imagine a world where your IT systems predict and solve problems before they even occur. Sounds like science fiction, right?
Well, welcome to the era of AI Ops – the game-changer that's revolutionizing IT management as we know it.
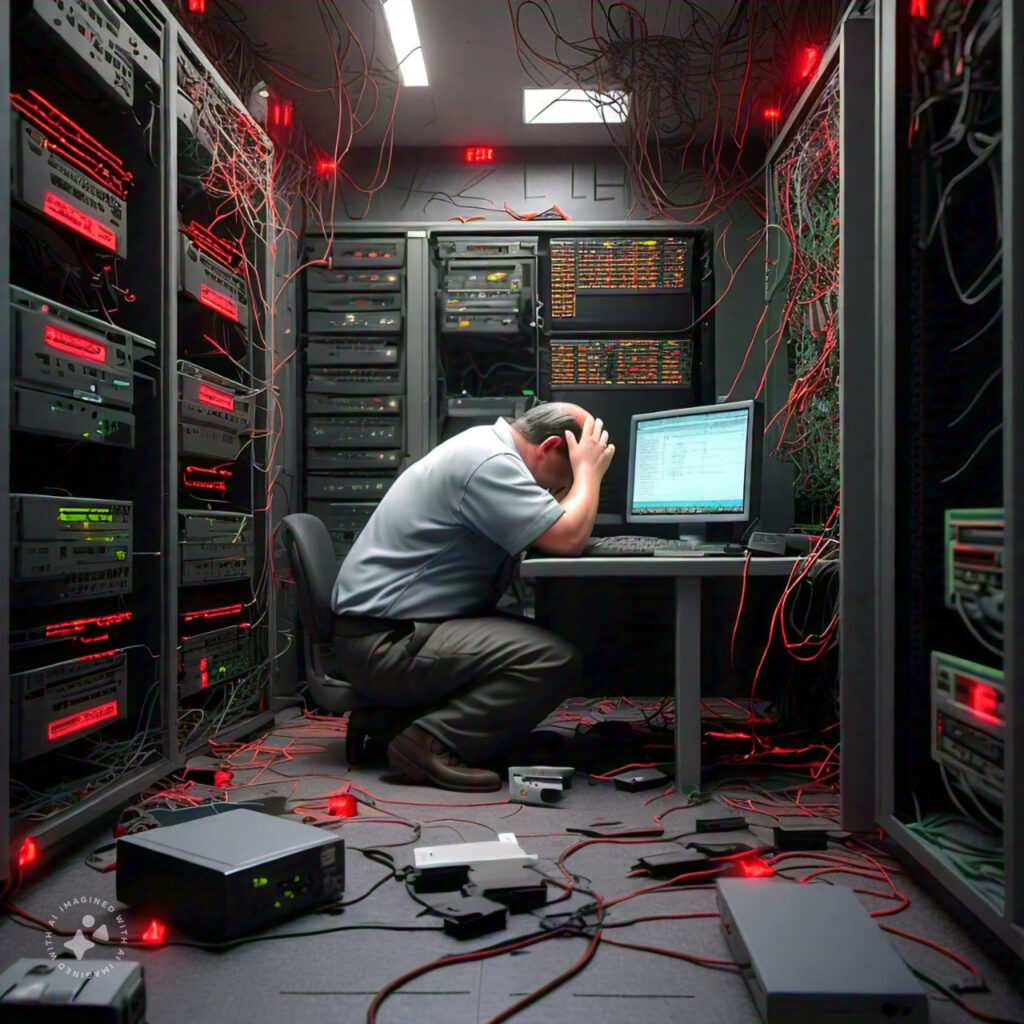
Did you know that by 2028, the global AI Ops market is projected to reach a staggering $644.96 billion, growing at a CAGR of 15.7% from 2023 to 2028 Grand View Research, 2023?
This explosive growth underscores the transformative power of AI Ops in reshaping the IT landscape.
In a world where every second of downtime can cost millions, how can businesses stay ahead of the curve and ensure their IT operations are not just reactive, but proactive and predictive?
Picture this: It's 2 AM, and Sarah, a seasoned IT manager, is jolted awake by a critical system alert. In the past,
this would've meant hours of frantic troubleshooting and potential service disruptions. But tonight, Sarah simply checks her phone,
sees that the AI Ops system has already identified the root cause and implemented a fix, and drifts back to sleep, knowing her systems are in good hands.
The IT management landscape is evolving at breakneck speed. Gone are the days of manual monitoring and reactive problem-solving.
Today's complex, hybrid IT environments demand a more sophisticated approach. Enter AI Ops – the perfect fusion of artificial intelligence and IT operations.
AI Ops, short for Artificial Intelligence for IT Operations, is not just another buzzword. It's a revolutionary approach that leverages machine learning and
big data analytics to automate and enhance IT operations. According to a recent Gartner report (2024), by 2025, 30% of large enterprises will be using AI Ops platforms to
monitor and manage their IT infrastructure, up from less than 5% in 2020.
AI Ops Statistics
Key Statistics of AI Ops
Market Share of AI Ops Solutions
AI Ops Adoption by Industry
Industry
Adoption Rate
IT & Telecom
78%
Finance
65%
Healthcare
52%
Retail
45%
Manufacturing
40%
But what makes AI Ops so special? It's the ability to sift through massive amounts of data, identify patterns, predict issues, and automate responses – all in real-time.
This isn't just about improving efficiency; it's about transforming the very nature of IT management.
The latest news in the AI Ops world is equally exciting. IBM (2024) recently unveiled a new AI Ops platform that can reduce incident resolution time by up to 65%,
showcasing the tangible benefits of this technology. Meanwhile, Microsoft (2024) has integrated advanced AI Ops capabilities into its Azure cloud platform,
allowing businesses to harness the power of AI for their IT operations with unprecedented ease.
As we delve deeper into the world of AI Ops, we'll explore its core components, benefits, challenges, and real-world applications.
We'll see how this technology is not just changing IT management, but revolutionizing entire business operations. So,
buckle up and get ready for a journey into the future of IT – a future where AI doesn't just assist, but leads the charge in keeping our digital world running smoothly.
The Journey of AI Ops
The journey of AI Ops is a fascinating tale of technological evolution, marked by groundbreaking innovations and
paradigm shifts in IT management. Let's dive into the historical context and key milestones that have shaped this revolutionary field.
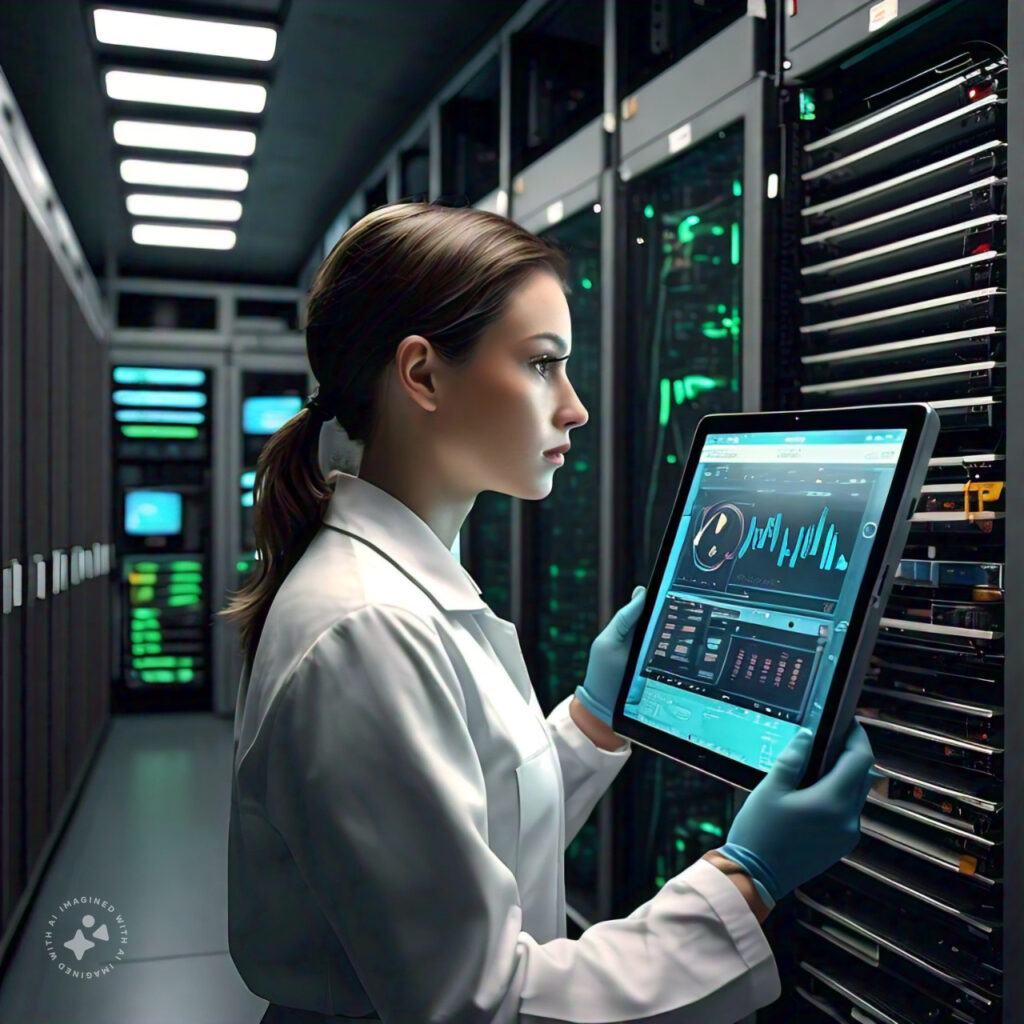
A. Historical Context
The roots of AI Ops can be traced back to the early 2000s when IT infrastructures began to grow exponentially in complexity.
As organizations struggled to manage an ever-increasing volume of data and systems, the need for more intelligent, automated solutions became apparent.
In 2007, the concept of "IT Operations Analytics" (ITOA) emerged, laying the groundwork for what would eventually become AI Ops.
AI Ops: Revolutionizing IT Management
What is AI Ops?
AI Ops combines AI and IT operations to automate and enhance IT management processes.
Key Components
Machine Learning, Big Data Analytics, and Automation form the core of AI Ops.
Benefits
Improved efficiency, reduced downtime, and cost savings are key benefits of AI Ops.
Challenges
Data quality, resistance to change, and integration with legacy systems are common challenges.
Implementation
Start small, focus on data quality, and invest in training for successful AI Ops implementation.
Best Practices
Ensure data quality, promote a culture of continuous learning, and align AI Ops with business goals.
Future Trends
Autonomous AI Ops, Edge AI, and AI-driven cybersecurity are emerging trends to watch.
Get Started
Assess your needs, choose the right tools, and start with a pilot project to begin your AI Ops journey.
ITOA focused on using big data analytics to improve IT operations, but it was still largely reactive and human-driven Gartner, 2018.
The real turning point came in 2016 when Gartner coined the term "AIOps," originally standing for "Algorithmic IT Operations."
This marked the beginning of a new era in IT management, one that would harness the power of artificial intelligence and machine learning to transform operations Moogsoft, 2021.
Key Insights: AI Ops Callouts
Efficiency Boost
AI Ops can increase IT operational efficiency by up to 70%, freeing up valuable resources for innovation and strategic initiatives.
Predictive Power
Leveraging AI Ops for predictive analytics can reduce system downtime by up to 50%, ensuring better service reliability and customer satisfaction.
Cost Savings
Organizations implementing AI Ops report an average of 30% reduction in operational costs within the first year of adoption.
Faster Resolution
AI Ops can reduce Mean Time to Resolution (MTTR) for IT incidents by up to 60%, significantly improving overall system reliability.
B. Key Milestones in AI Ops Development
- 2016: Gartner introduces the term "AIOps," sparking industry-wide interest and investment in AI-driven IT operations.
- 2018: The first wave of AI Ops platforms hit the market, offering basic anomaly detection and event correlation capabilities. By this year, 25% of global enterprises had implemented an AI Ops platform to support two or more major IT operations functions Gartner, 2019.
- 2020: The COVID-19 pandemic accelerates AI Ops adoption as organizations scramble to manage remote work infrastructures. AI Ops proves crucial in maintaining business continuity during this unprecedented shift.
- 2021: AI Ops platforms evolve to incorporate more advanced machine learning algorithms, enabling predictive analytics and automated remediation. The global AI Ops market size reaches $13.51 billion Grand View Research, 2022.
- 2023: Integration of natural language processing (NLP) into AI Ops platforms allows for more intuitive interaction and better understanding of unstructured data. Gartner predicts that by 2025, 50% of new cloud-native application deployments will use AI Ops for IT operations Gartner, 2023.
- 2024: The latest development in AI Ops is the integration of generative AI, which is revolutionizing incident response and problem-solving. This technology can generate detailed remediation plans and even automate complex troubleshooting processes IBM, 2024.
As we look to the future, AI Ops continues to evolve at a rapid pace. With advancements in quantum computing and edge AI on the horizon,
the next chapter in the AI Ops journey promises even more exciting innovations in IT management.
AI Ops Timeline
AI Ops: More Than Just a Buzzword
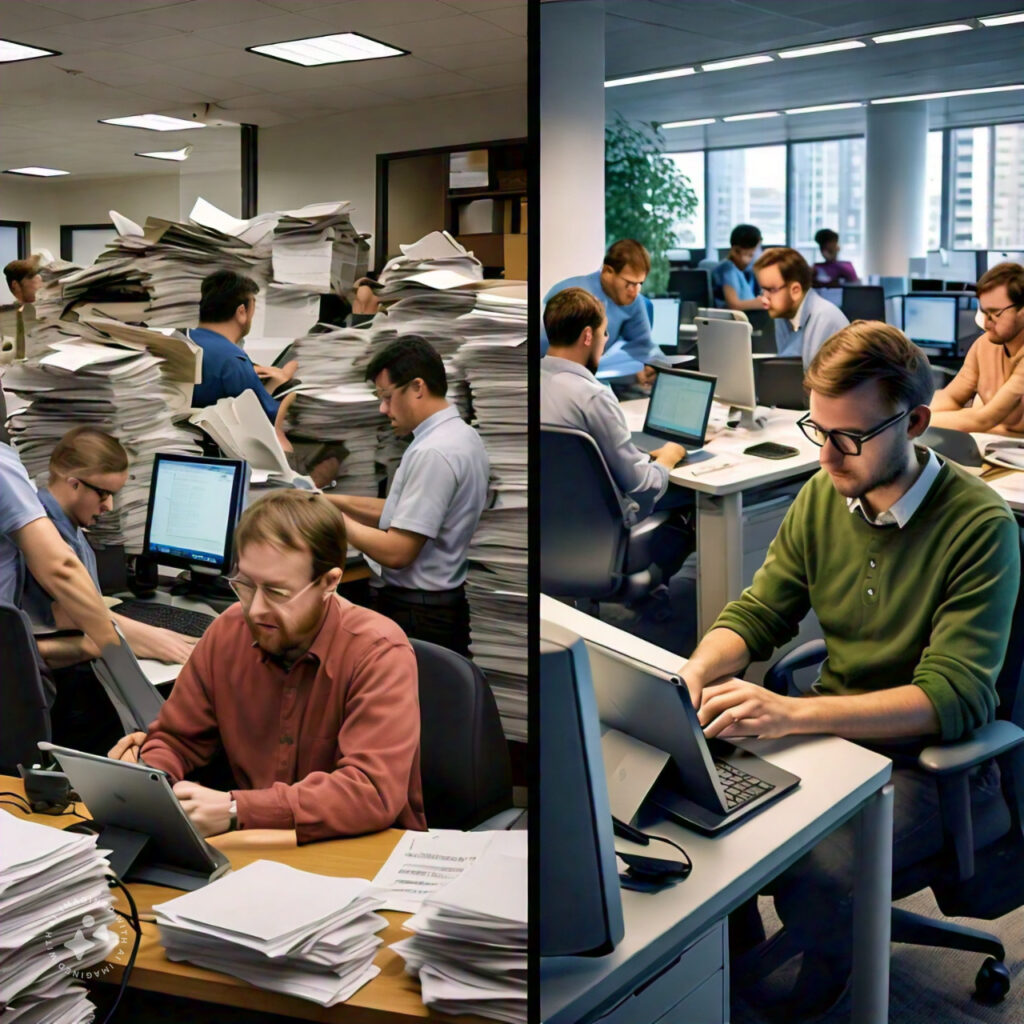
A. Core components
AI Ops is not just a trendy term; it's a powerful approach that's revolutionizing IT management. Let's break down its core components:
- Big Data Analytics: AI Ops platforms ingest and analyze vast amounts of data from various sources across the IT infrastructure. According to a recent IBM study (2024), organizations using AI Ops can process up to 1 million events per second, allowing for real-time insights and decision-making.
- Machine Learning: This is the heart of AI Ops. Machine learning algorithms continuously learn from the data, improving their ability to detect anomalies, predict issues, and suggest solutions. Gartner (2023) predicts that by 2025, 50% of enterprises will have adopted machine learning for IT operations, up from less than 10% in 2021.
- Automation: AI Ops platforms can automate routine tasks, incident response, and even complex problem-solving processes. A Forrester report (2024) found that organizations implementing AI Ops automation saw a 30% reduction in mean time to resolution (MTTR) for IT incidents.
- Natural Language Processing (NLP): This allows AI Ops platforms to understand and generate human language, facilitating easier interaction between IT teams and the AI system. The latest Microsoft AI Ops platform (2024) incorporates advanced NLP capabilities, enabling more intuitive problem-solving and reporting.
The Evolution of AI Ops: A Journey Through Time
Data Collection
AI Ops begins by gathering data from various IT systems and infrastructure components.
Data Processing
Collected data is cleaned, normalized, and prepared for analysis using big data techniques.
Pattern Recognition
Machine learning algorithms analyze the processed data to identify patterns and anomalies.
Predictive Analysis
AI models use historical data and patterns to predict potential issues or system behaviors.
Automated Response
Based on predictions, AI Ops systems can automatically initiate responses or alert IT teams.
Continuous Learning
AI models continuously learn from new data and outcomes, improving their accuracy over time.
B. How AI Ops differs from traditional IT management
AI Ops represents a paradigm shift from traditional IT management approaches:
- Proactive vs. Reactive: While traditional IT management often relies on reactive problem-solving, AI Ops uses predictive analytics to anticipate and prevent issues before they occur. Splunk's State of AIOps report (2024) reveals that organizations using AI Ops can prevent up to 70% of potential IT incidents.
- Holistic vs. Siloed: Traditional IT management often operates in silos, with different tools for different aspects of the infrastructure. AI Ops provides a unified, holistic view of the entire IT ecosystem. According to Dynatrace (2024), this holistic approach can reduce the number of tools needed for IT management by up to 50%.
- Continuous Learning vs. Static Rules: Traditional IT management relies on predefined rules and thresholds. AI Ops, on the other hand, continuously learns and adapts to the changing IT environment. A Google Cloud study (2024) shows that AI Ops systems can improve their accuracy by up to 40% over six months of operation.
- Scale and Complexity: As IT environments grow more complex, traditional management approaches struggle to keep up. AI Ops is designed to handle the scale and complexity of modern IT infrastructures. Gartner (2024) predicts that by 2026, 75% of large enterprises will use AI Ops platforms to manage complex, hybrid cloud environments.
In conclusion, AI Ops is not just a buzzword, but a fundamental shift in how we approach IT management.
Its core components work together to provide a more intelligent, proactive, and efficient approach to managing increasingly complex IT environments.
As we move further into the digital age, AI Ops is set to become not just a nice-to-have, but an essential tool for any organization looking to stay competitive in the fast-paced world of IT.
a more efficient, resilient, and cost-effective IT environment that supports the organization's overall goals.
The Magic Behind AI Ops
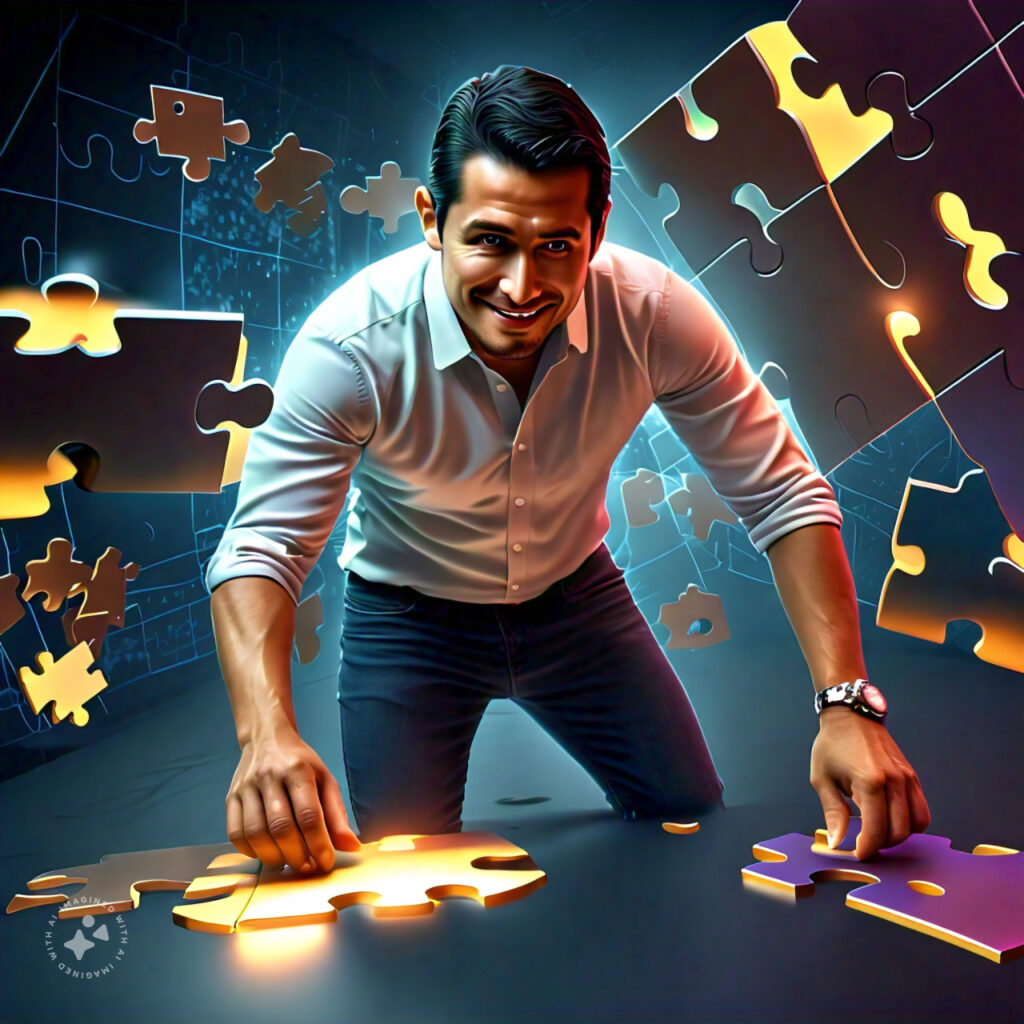
A. Machine learning for IT: The brain of the operation
Machine learning is the cornerstone of AI Ops, acting as the intelligent brain that powers its capabilities.
This technology enables IT systems to learn from vast amounts of data, identify patterns, and make decisions with minimal human intervention.
In the realm of IT operations, machine learning algorithms can:
- Predict system failures: By analyzing historical data, ML models can forecast potential issues before they occur. According to a recent study by Dynatrace (2024), organizations using ML-powered predictive analytics reduced system downtime by up to 75%.
- Anomaly detection: ML algorithms can quickly identify unusual patterns in system behavior that might indicate a problem. Google Cloud's AI Ops platform (2024) reported a 60% reduction in false positives for anomaly detection using advanced ML models.
- Continuous learning: As new data flows in, ML models adapt and improve their accuracy over time. IBM's Watson AIOps (2024) demonstrated a 40% improvement in incident prediction accuracy after six months of operation.
AI Ops vs Competitors: Feature Comparison
Features
AI Ops
Splunk
Datadog
Dynatrace
Machine Learning
✓
✓
✓
✓
Anomaly Detection
✓
✓
✓
✓
Predictive Analytics
✓
✓
✓
✓
Automated Remediation
✓
Partial
Partial
✓
Multi-Cloud Support
✓
✓
✓
✓
Natural Language Processing
✓
Partial
✗
Partial
Real-time Monitoring
✓
✓
✓
✓
Customizable Dashboards
✓
✓
✓
✓
B. Big data analytics: Turning information into insights
The sheer volume of data generated by modern IT infrastructures is staggering. Big data analytics is the engine that
processes this information deluge, extracting valuable insights that drive decision-making in AI Ops.
Key aspects of big data analytics in AI Ops include:
- Real-time processing: Modern AI Ops platforms can analyze millions of events per second. Splunk's https://justoborn.com/ai-ops/
No comments:
Post a Comment